Closing the AI generalization gap by adjusting for dermatology condition distribution differences across clinical settings
CoRR(2024)
摘要
Recently, there has been great progress in the ability of artificial
intelligence (AI) algorithms to classify dermatological conditions from
clinical photographs. However, little is known about the robustness of these
algorithms in real-world settings where several factors can lead to a loss of
generalizability. Understanding and overcoming these limitations will permit
the development of generalizable AI that can aid in the diagnosis of skin
conditions across a variety of clinical settings. In this retrospective study,
we demonstrate that differences in skin condition distribution, rather than in
demographics or image capture mode are the main source of errors when an AI
algorithm is evaluated on data from a previously unseen source. We demonstrate
a series of steps to close this generalization gap, requiring progressively
more information about the new source, ranging from the condition distribution
to training data enriched for data less frequently seen during training. Our
results also suggest comparable performance from end-to-end fine tuning versus
fine tuning solely the classification layer on top of a frozen embedding model.
Our approach can inform the adaptation of AI algorithms to new settings, based
on the information and resources available.
更多查看译文
AI 理解论文
溯源树
样例
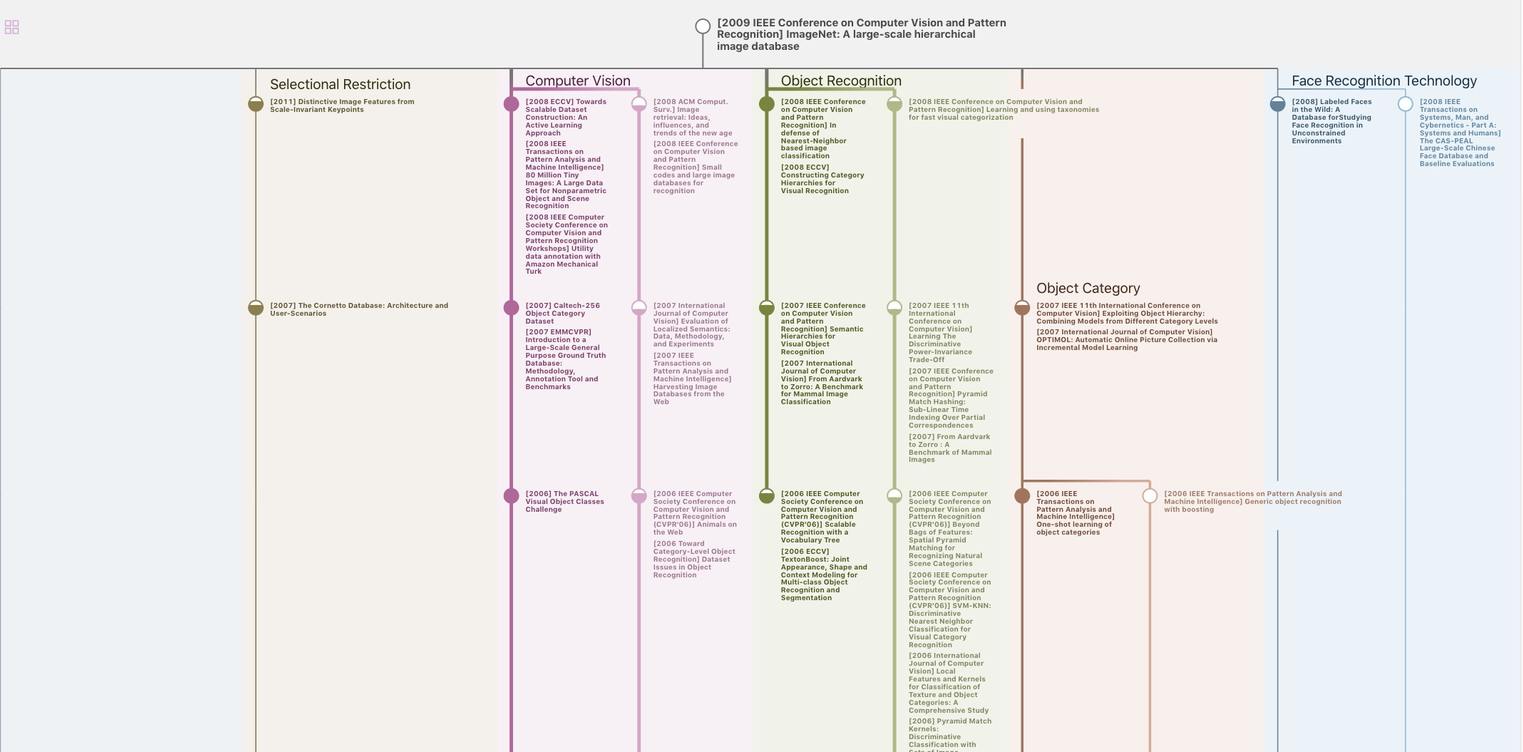
生成溯源树,研究论文发展脉络
Chat Paper
正在生成论文摘要