A Cross-Layered Cluster Embedding Learning Network with Regularization for Multivariate Time Series Anomaly Detection
The Journal of Supercomputing(2023)
Hunan Normal University | Providence University
Abstract
The devices deployed across diverse industrial scenarios have generated significant network traffic related to time. The system’s irregular operation could result in substantial bad influence. Anomaly detection technologies utilized for identifying possible non-standard behaviours are paramount; furthermore, multivariate time series exhibit complex dependencies besides temporal correlation. However, most previous methods merely consider the temporal and variable correlation of time series data, neglecting the distance metrics among the sequences, leading to a deficiency in the model’s anomaly detection ability. We propose a multivariate time series anomaly detection model based on the encoder–decoder architecture (CCER-ED). The model considers the similarity measure between temporal subsequences and designs a multi-scale feature embedding module for leveraging more interrelated properties. Moreover, the interrelations among sensors are explicitly learned using a manifold regularization graph structure. On this basis, an improved data fusion approach based on a multi-head self-attention mechanism is designed for capturing global feature representation, effectively integrating various aspects of information. Evaluations using the real-world datasets SWAT and WADI and performance analysis show that the proposed approach achieves improvement over the baselines in the recall and F1-score of anomaly detection performance at 9.3% and 8.5% (maximum), respectively, outperforming other existing methods.
MoreTranslated text
Key words
Anomaly detection,Multivariate time series,Graph structure,Cluster embedding,Attention mechanism
求助PDF
上传PDF
View via Publisher
AI Read Science
AI Summary
AI Summary is the key point extracted automatically understanding the full text of the paper, including the background, methods, results, conclusions, icons and other key content, so that you can get the outline of the paper at a glance.
Example
Background
Key content
Introduction
Methods
Results
Related work
Fund
Key content
- Pretraining has recently greatly promoted the development of natural language processing (NLP)
- We show that M6 outperforms the baselines in multimodal downstream tasks, and the large M6 with 10 parameters can reach a better performance
- We propose a method called M6 that is able to process information of multiple modalities and perform both single-modal and cross-modal understanding and generation
- The model is scaled to large model with 10 billion parameters with sophisticated deployment, and the 10 -parameter M6-large is the largest pretrained model in Chinese
- Experimental results show that our proposed M6 outperforms the baseline in a number of downstream tasks concerning both single modality and multiple modalities We will continue the pretraining of extremely large models by increasing data to explore the limit of its performance
Upload PDF to Generate Summary
Must-Reading Tree
Example
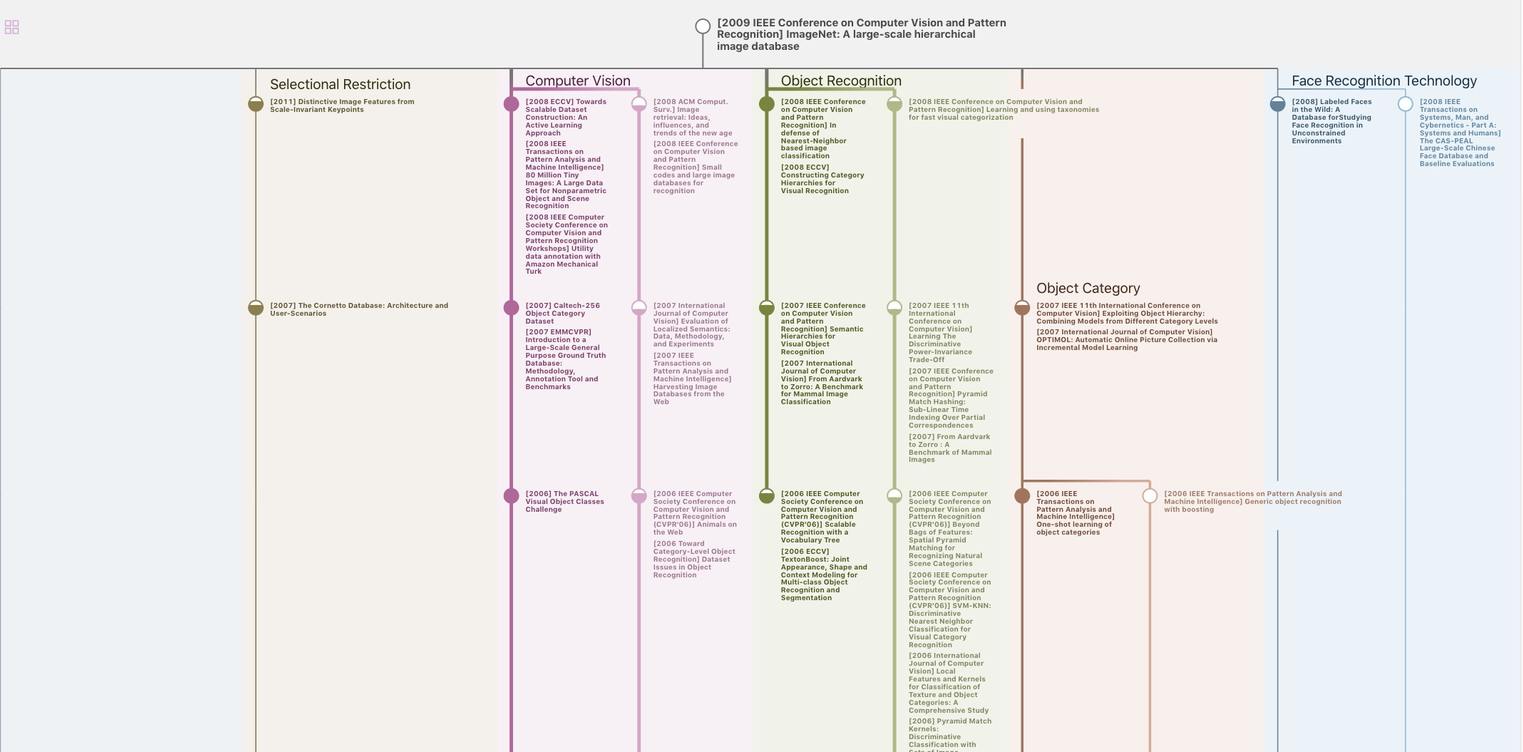
Generate MRT to find the research sequence of this paper
Related Papers
2003
被引用1552 | 浏览
2020
被引用17 | 浏览
2023
被引用85 | 浏览
2023
被引用6 | 浏览
2021
被引用31 | 浏览
2022
被引用28 | 浏览
2022
被引用57 | 浏览
2022
被引用83 | 浏览
2022
被引用18 | 浏览
2023
被引用118 | 浏览
2023
被引用29 | 浏览
2023
被引用6 | 浏览
2023
被引用20 | 浏览
Data Disclaimer
The page data are from open Internet sources, cooperative publishers and automatic analysis results through AI technology. We do not make any commitments and guarantees for the validity, accuracy, correctness, reliability, completeness and timeliness of the page data. If you have any questions, please contact us by email: report@aminer.cn
Chat Paper