XEmoAccent: Embracing Diversity in Cross-Accent Emotion Recognition Using Deep Learning
IEEE ACCESS(2024)
摘要
Speech is a powerful means to expressing thoughts, emotions, and perspectives. However, accurately determining the emotions conveyed through speech remains a challenging task. Existing manual methods for analyzing speech to recognize emotions are prone to errors, limiting our understanding and response to individuals’ emotional states. To address diverse accents, an automated system capable of real-time emotion prediction from human speech is needed. This paper introduces a speech emotion recognition (SER) system that leverages supervised learning techniques to tackle cross-accent diversity. Distinctively, the system extracts a comprehensive set of nine speech features—Zero Crossing Rate, Mel Spectrum, Pitch, Root Mean Square values, Mel Frequency Cepstral Coefficients, chroma-stft, and three spectral features (Centroid, Contrast, and Roll-off) for refined speech signal processing and recognition. Seven machine learning models are employed, encompassing Random Forest, Logistic Regression, Decision Tree, Support Vector Machines, Gaussian Naive Bayes, K-Nearest Neighbors, ensemble learning, and four individual, hybrid deep learning models including Long short-term memory (LSTM) and 1-Dimensional Convolutional Neural Network (1D-CNN) with stratified cross-validation. Audio samples from diverse English regions are combined to train the models. The performance evaluation results of conventional machine learning and deep learning models indicate that the Random Forest-based feature selection model achieves the highest accuracy of up to 76% among the conventional machine learning models. Simultaneously, the 1D-CNN model with stratified cross-validation reaches up to 99% accuracy. The proposed framework enhances the cross-accent emotion recognition accuracy up to 86.3%, 89.87%, 90.27%, and 84.96% by margins of 14.71%, 10.15%, 9.6%, and 16.52% respectively.
更多查看译文
关键词
Machine learning,Deep learning,Speech Emotion Recognition (SER),Random Forest (RF),Logistic Regression (LR),Decision Tree (DT),Support Vector Machines (SVM),K-Nearest Neighbors (KNN),1-Dimensional Convolutional Neural Network (1D-CNN)
AI 理解论文
溯源树
样例
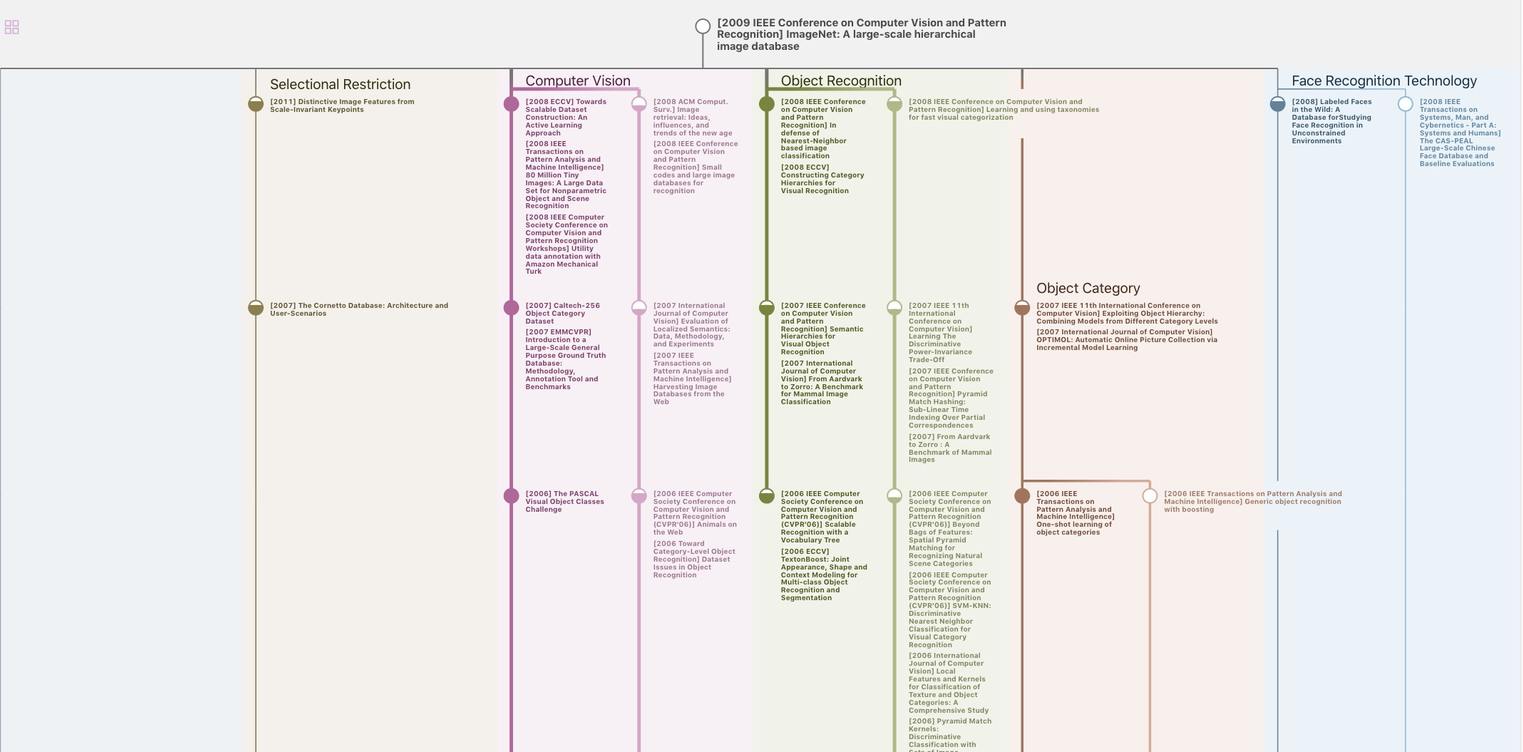
生成溯源树,研究论文发展脉络
Chat Paper
正在生成论文摘要