Multirate Progressive Entropy Model for Learned Image Compression
IEEE Transactions on Circuits and Systems for Video Technology(2024)
摘要
This paper proposes a unified and efficient entropy coding method for learned image compression (LIC) from the perspective of traditional signal processing. First, the consistency of structures and optimization objectives are used to interpret the existing split-coded-then-merge entropy coding strategies in LIC as a particular filter banks framework, with feature separation and feature aggregation representing the analysis filter bank and synthesis filter bank, respectively. Thus, we borrow the design from the multirate filter banks and proposed Multirate Progressive Entropy Model (MPEM) to enhance the rate-distortion performance and decoding speed. In particular, we create an analysis filter bank that divides compact features into a few nonuniform subsets based on various spatial and channel sampling rates. Then multi-scale detail and mean coefficients within the current subset are used as prior representations to help generate the prediction parameters of the next subset, and the carefully designed synthetic filter bank performs a near-perfect reconstruction of the features. In addition, we propose a Multi-level Edge Attention Moudal (MEAM) to increase the edge and texture information’s contribution and reduce the high-frequency information loss brought on by MPEM’s inherent multi-rate spatial sampling, which leverages the edge operator and structural reparameterization principles. The results of the experiments show that, in comparison to the effective LIC methods and traditional code, the proposed MPEM can decode data at a cutting-edge speed while also offering comparable rate-distortion performance.
更多查看译文
关键词
Deep learning,image compression,multi-rate filter bank,entropy model,attention mechanism
AI 理解论文
溯源树
样例
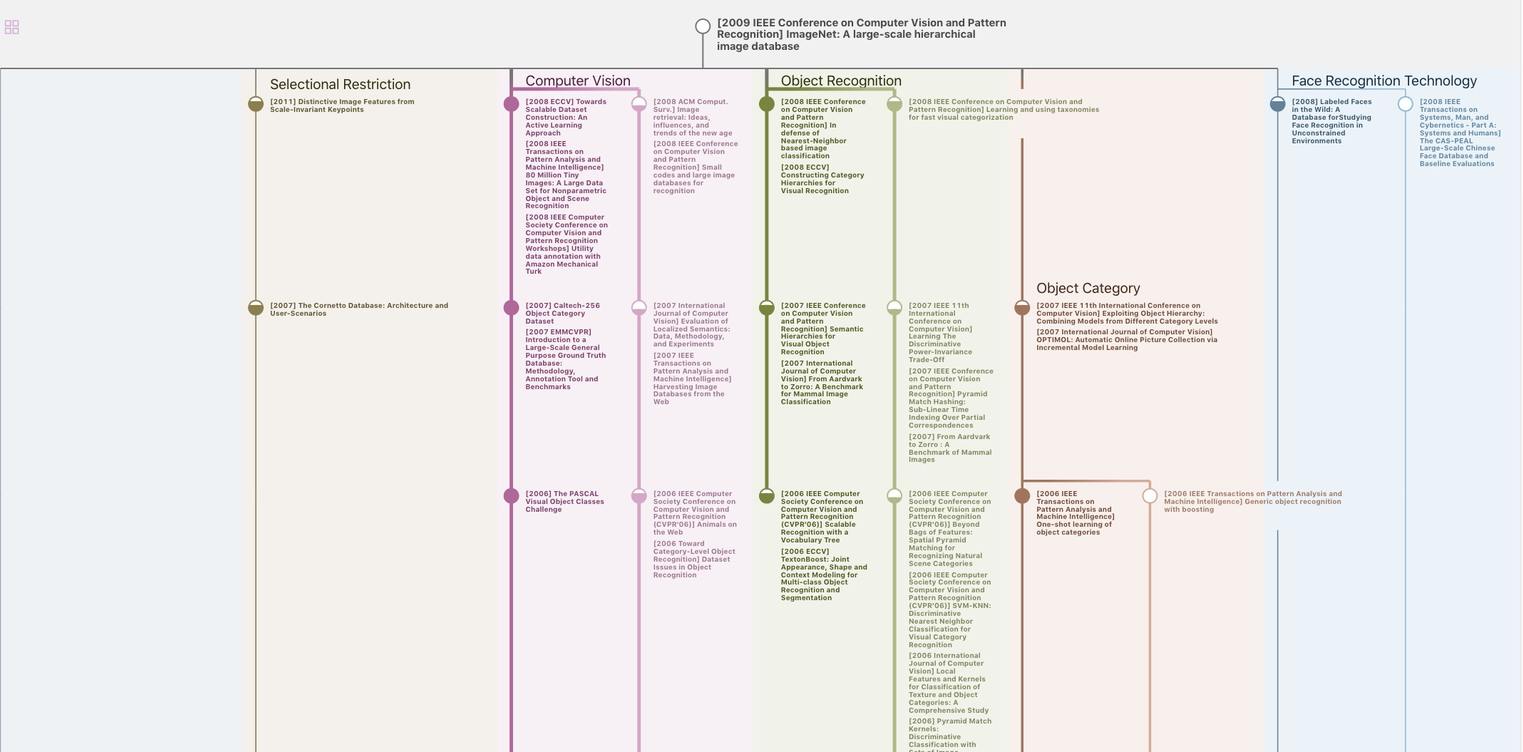
生成溯源树,研究论文发展脉络
Chat Paper
正在生成论文摘要