Reusable Architecture Growth for Continual Stereo Matching.
IEEE transactions on pattern analysis and machine intelligence(2024)
摘要
The remarkable performance of recent stereo depth estimation models benefits from the successful use of convolutional neural networks to regress dense disparity. Akin to most tasks, this needs gathering training data that covers a number of heterogeneous scenes at deployment time. However, training samples are typically acquired continuously in practical applications, making the capability to learn new scenes continually even more crucial. For this purpose, we propose to perform continual stereo matching where a model is tasked to 1) continually learn new scenes, 2) overcome forgetting previously learned scenes, and 3) continuously predict disparities at inference. We achieve this goal by introducing a Reusable Architecture Growth (RAG) framework. RAG leverages task-specific neural unit search and architecture growth to learn new scenes continually in both supervised and self-supervised manners. It can maintain high reusability during growth by reusing previous units while obtaining good performance. Additionally, we present a Scene Router module to adaptively select the scene-specific architecture path at inference. Comprehensive experiments on numerous datasets show that our framework performs impressively in various weather, road, and city circumstances and surpasses the state-of-the-art methods in more challenging cross-dataset settings. Further experiments also demonstrate the adaptability of our method to unseen scenes, which can facilitate end-to-end stereo architecture learning and practical deployment.
更多查看译文
关键词
Stereo Matching,Deep Learning,Continual Learning,Domain Adaptation,Architecture Growth
AI 理解论文
溯源树
样例
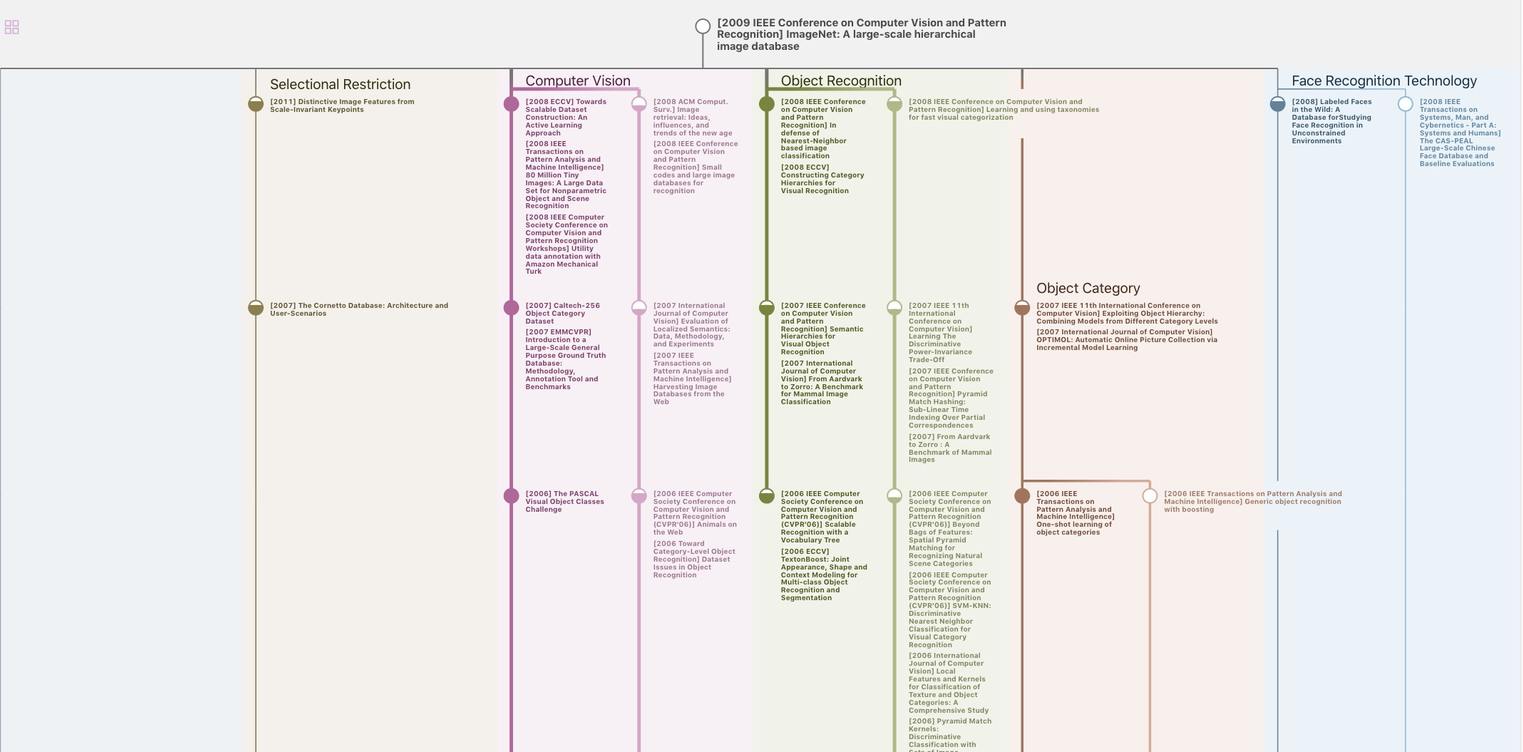
生成溯源树,研究论文发展脉络
Chat Paper
正在生成论文摘要