Adaptive Uncertainty-Based Learning for Text-Based Person Retrieval
AAAI 2024(2024)
摘要
Text-based person retrieval aims at retrieving a specific pedestrian image from a gallery based on textual descriptions. The primary challenge is how to overcome the inherent heterogeneous modality gap in the situation of significant intra-class variation and minimal inter-class variation. Existing approaches commonly employ vision-language pre-training or attention mechanisms to learn appropriate cross-modal alignments from noise inputs. Despite commendable progress, current methods inevitably suffer from two defects: 1) Matching ambiguity, which mainly derives from unreliable matching pairs; 2) One-sided cross-modal alignments, stemming from the absence of exploring one-to-many correspondence, i.e., coarse-grained semantic alignment. These critical issues significantly deteriorate retrieval performance. To this end, we propose a novel framework termed Adaptive Uncertainty-based Learning (AUL) for text-based person retrieval from the uncertainty perspective. Specifically, our AUL framework consists of three key components: 1) Uncertainty-aware Matching Filtration that leverages Subjective Logic to effectively mitigate the disturbance of unreliable matching pairs and select high-confidence cross-modal matches for training; 2) Uncertainty-based Alignment Refinement, which not only simulates coarse-grained alignments by constructing uncertainty representations but also performs progressive learning to incorporate coarse- and fine-grained alignments properly; 3) Cross-modal Masked Modeling that aims at exploring more comprehensive relations between vision and language. Extensive experiments demonstrate that our AUL method consistently achieves state-of-the-art performance on three benchmark datasets in supervised, weakly supervised, and domain generalization settings. Our code is available at https://github.com/CFM-MSG/Code-AUL.
更多查看译文
关键词
CV: Image and Video Retrieval,CV: Language and Vision,ML: Calibration & Uncertainty Quantification
AI 理解论文
溯源树
样例
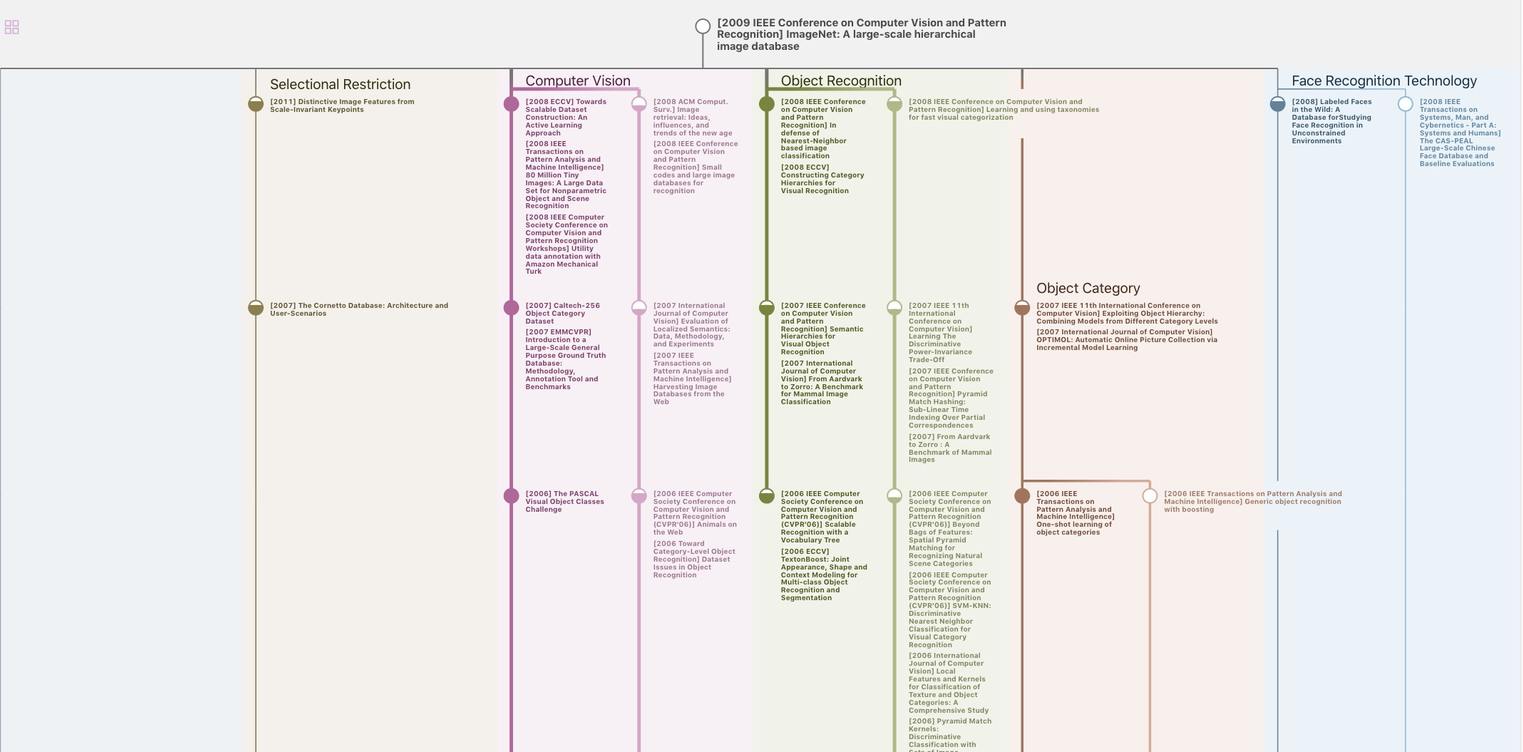
生成溯源树,研究论文发展脉络
Chat Paper
正在生成论文摘要