Generalized Maximum Entropy Differential Dynamic Programming
arxiv(2024)
摘要
We present a sampling-based trajectory optimization method derived from the
maximum entropy formulation of Differential Dynamic Programming with Tsallis
entropy. This method can be seen as a generalization of the legacy work with
Shannon entropy, which leads to a Gaussian optimal control policy for
exploration during optimization. With the Tsallis entropy, the optimal control
policy takes the form of q-Gaussian, which further encourages exploration
with its heavy-tailed shape. Moreover, in our formulation, the exploration
variance, which was scaled by a fixed constant inverse temperature in the
original formulation with Shannon entropy, is automatically scaled based on the
value function of the trajectory. Due to this property, our algorithms can
promote exploration when necessary, that is, the cost of the trajectory is
high, rather than using the same scaling factor. The simulation results
demonstrate the properties of the proposed algorithm described above.
更多查看译文
AI 理解论文
溯源树
样例
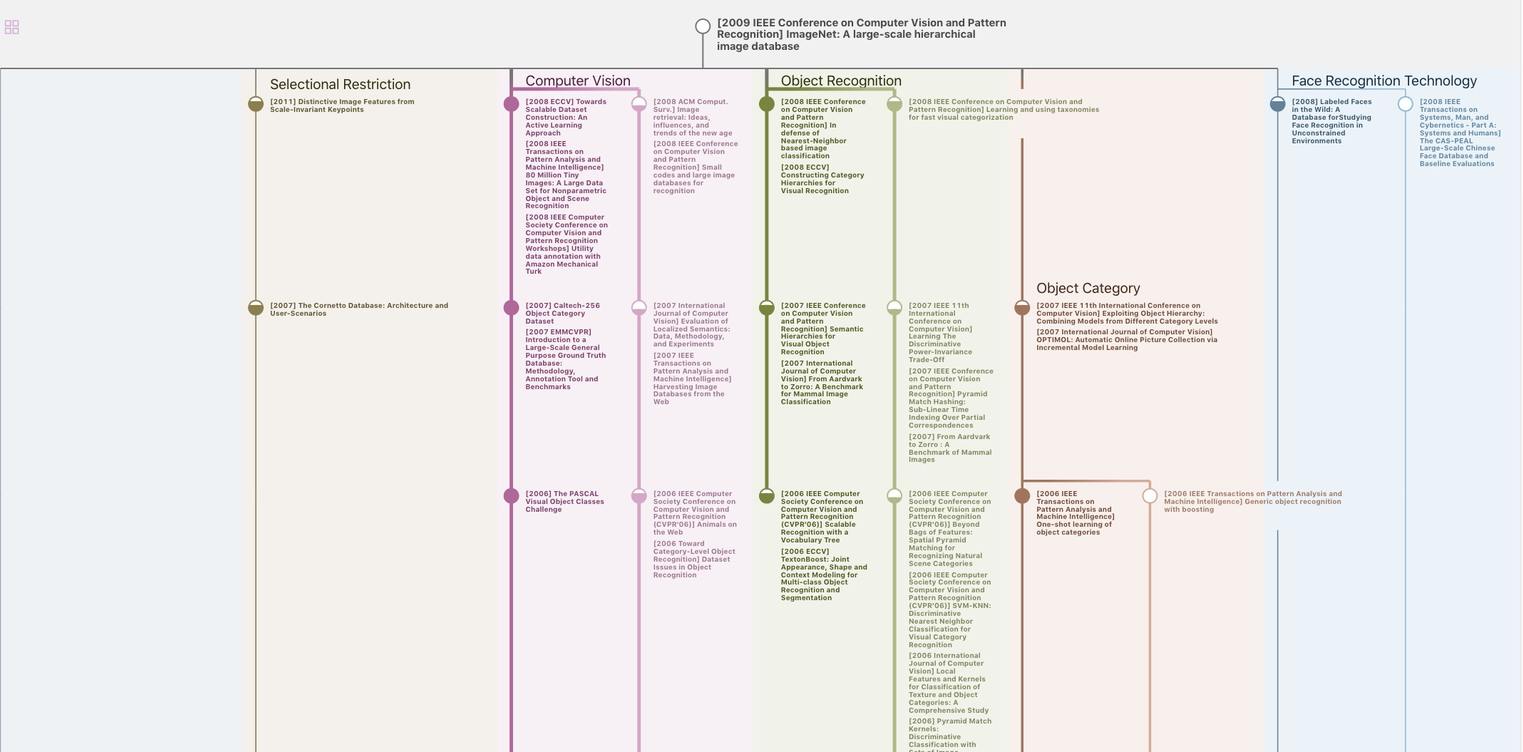
生成溯源树,研究论文发展脉络
Chat Paper
正在生成论文摘要