Multi-objective Optimization of Hybrid Energy Systems Based on Life Cycle Exergy and Economic Criteria
Energy and Built Environment(2024)
关键词
Hybrid energy system, Life cycle Exergy, Optimization,Renewable energy, Sustainability
AI 理解论文
溯源树
样例
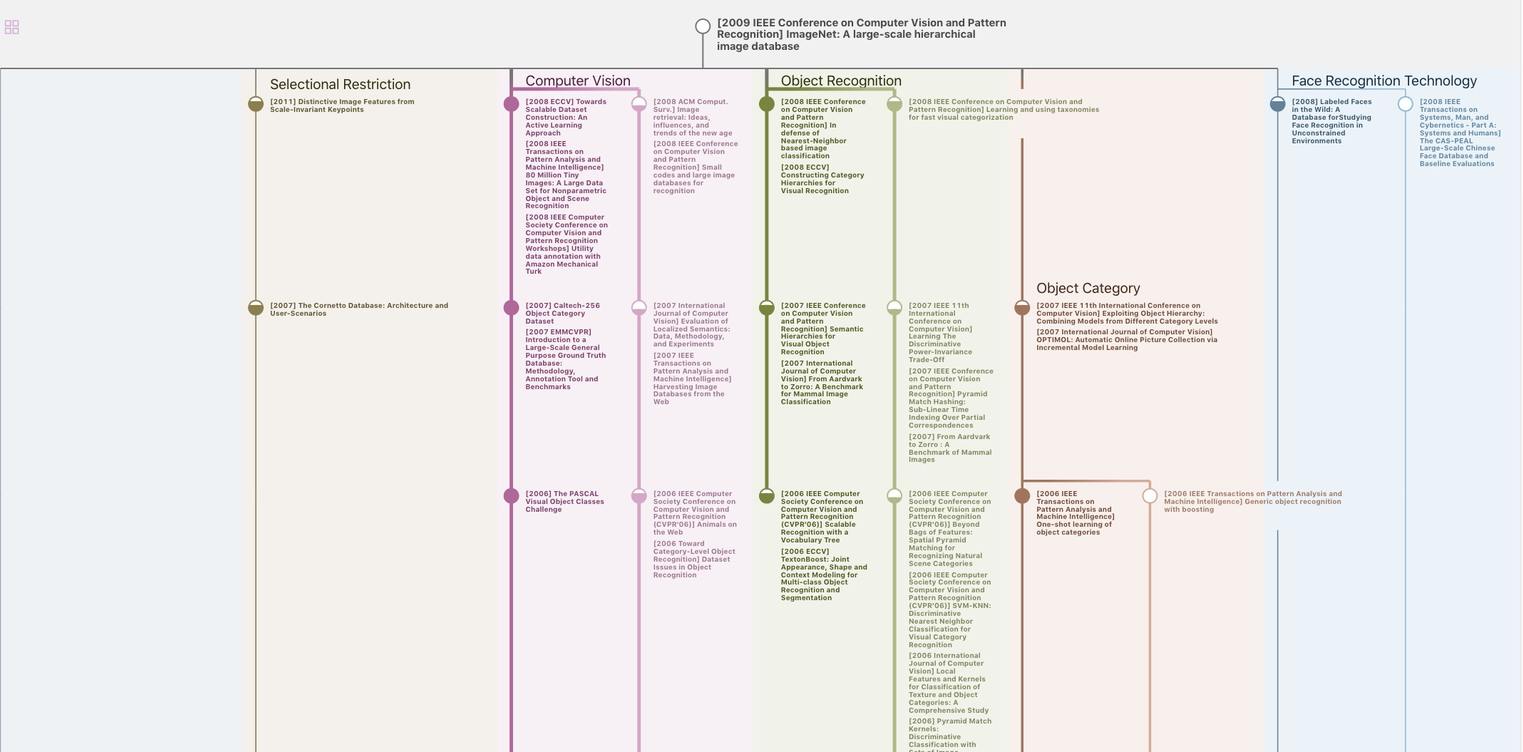
生成溯源树,研究论文发展脉络
Chat Paper
正在生成论文摘要