A New Information Complexity Measure for Multi-pass Streaming with Applications
CoRR(2024)
Abstract
We introduce a new notion of information complexity for multi-pass streaming
problems and use it to resolve several important questions in data streams.
In the coin problem, one sees a stream of n i.i.d. uniform bits and one
would like to compute the majority with constant advantage. We show that any
constant pass algorithm must use Ω(log n) bits of memory, significantly
extending an earlier Ω(log n) bit lower bound for single-pass
algorithms of Braverman-Garg-Woodruff (FOCS, 2020). This also gives the first
Ω(log n) bit lower bound for the problem of approximating a counter up
to a constant factor in worst-case turnstile streams for more than one pass.
In the needle problem, one either sees a stream of n i.i.d. uniform samples
from a domain [t], or there is a randomly chosen needle α∈[t] for
which each item independently is chosen to equal α with probability p,
and is otherwise uniformly random in [t]. The problem of distinguishing these
two cases is central to understanding the space complexity of the frequency
moment estimation problem in random order streams. We show tight multi-pass
space bounds for this problem for every p < 1/√(n log^3 n), resolving an
open question of Lovett and Zhang (FOCS, 2023); even for 1-pass our bounds
are new. To show optimality, we improve both lower and upper bounds from
existing results.
Our information complexity framework significantly extends the toolkit for
proving multi-pass streaming lower bounds, and we give a wide number of
additional streaming applications of our lower bound techniques, including
multi-pass lower bounds for ℓ_p-norm estimation, ℓ_p-point query and
heavy hitters, and compressed sensing problems.
MoreTranslated text
AI Read Science
Must-Reading Tree
Example
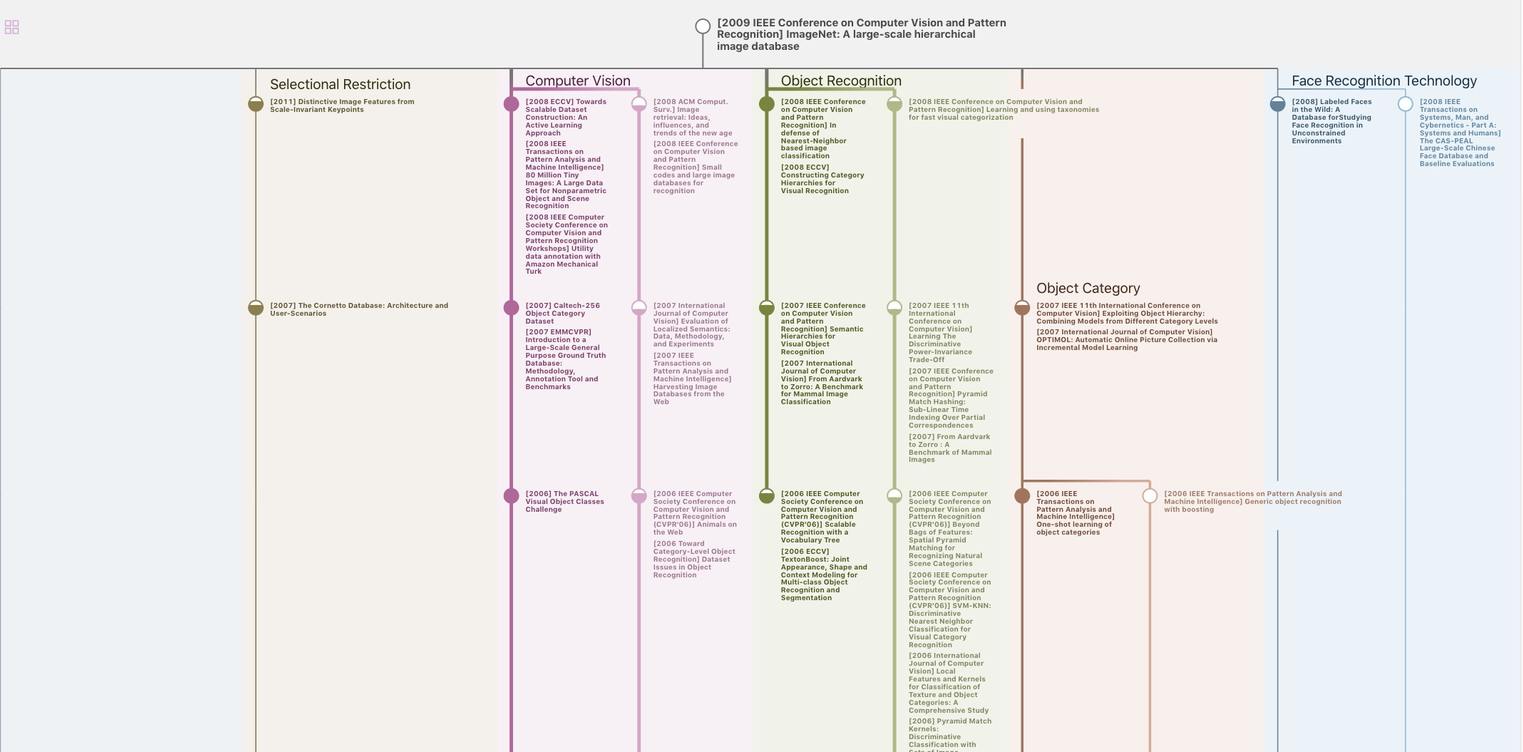
Generate MRT to find the research sequence of this paper
Chat Paper
Summary is being generated by the instructions you defined