Attention-Based Learning for Predicting Drug-Drug Interactions in Knowledge Graph Embedding Based on Multisource Fusion Information
INTERNATIONAL JOURNAL OF INTELLIGENT SYSTEMS(2024)
摘要
Drug combinations can reduce drug resistance and side effects and enable the improvement of disease treatment efficacy. Therefore, how to effectively identify drug-drug interactions (DDIs) is a challenging problem. Currently, there exist several approaches that leverage advanced representation learning and graph-based techniques for DDIs prediction. While these methods have demonstrated promising results, a limited number of approaches effectively utilize the potential of knowledge graphs (KGs), which provide information on drug attributes and multirelation among entities. In this work, we introduce a novel attention-based KGs representation learning framework. To encode drug SMILES sequence, a pretrained model is used, while molecular structure information is mapped as the initialization of nodes within the KG using a message-passing neural network. Additionally, the knowledge-aware graph attention network is employed to capture the drug and its topological neighbor representation in the KG representation module. To prevent the oversmoothing problem, the residual layer is used in the DDI prediction module. Comprehensive experiments on several datasets have demonstrated that the proposed method outperforms the state-of-the-art algorithms on the DDI prediction task across a range of evaluation metrics. It achieves an accuracy of 0.924 and an AUC of 0.9705 on the KEGG dataset and attains an ACC of 0.9777 and an AUC of 0.9959 on the OGB-biokg dataset. These experimental findings affirm that our approach is a dependable model for predicting the association of drugs.
更多查看译文
AI 理解论文
溯源树
样例
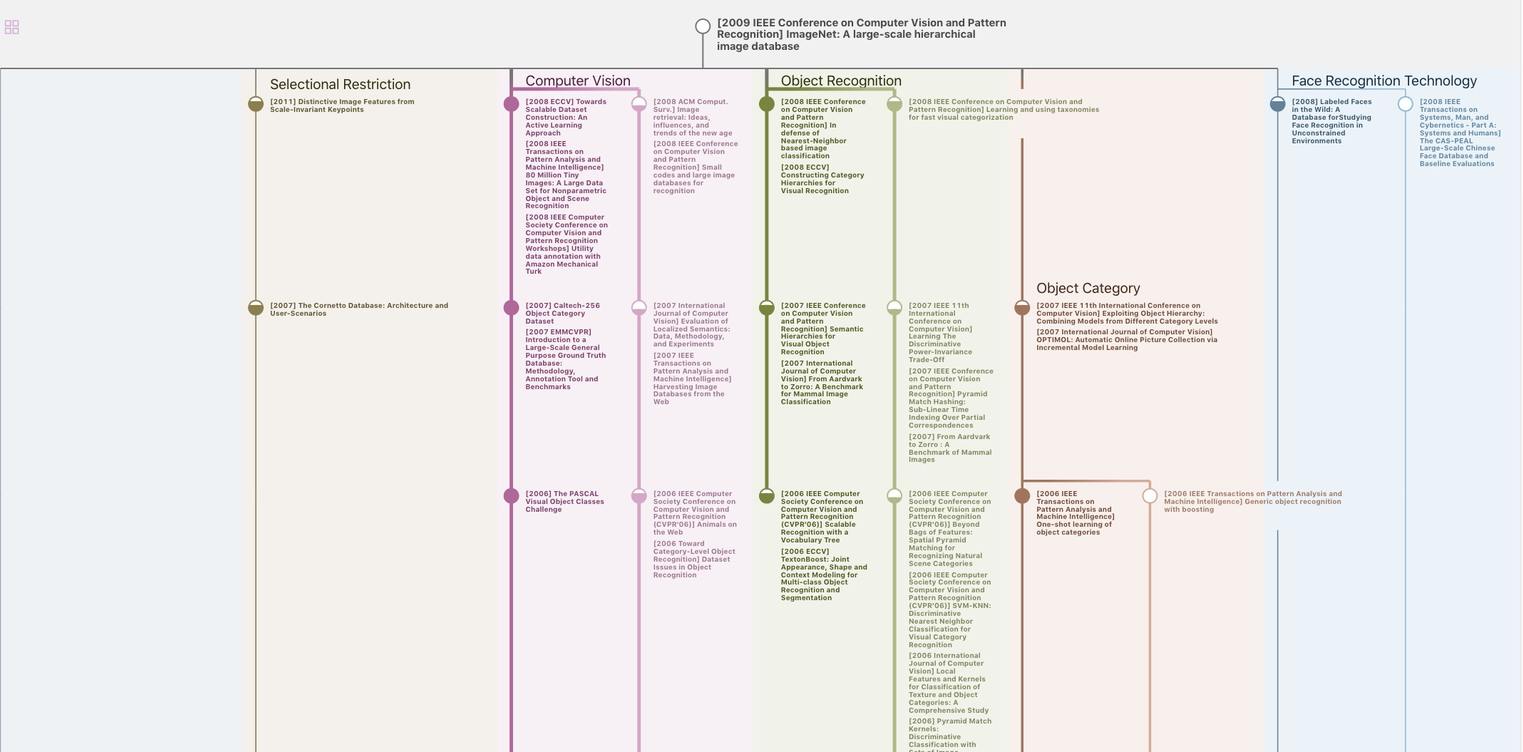
生成溯源树,研究论文发展脉络
Chat Paper
正在生成论文摘要