Few-shot Action Recognition via Multi-view Representation Learning
IEEE Transactions on Circuits and Systems for Video Technology(2024)
摘要
Few-shot action recognition aims to recognize novel action classes with limited labeled samples and has recently received increasing attention. The core objective of few-shot action recognition is to enhance the discriminability of feature representations. In this paper, we propose a novel multi-view representation learning network (MRLN) to model intra-video and inter-video relations for few-shot action recognition. Specifically, we first propose a spatial-aware aggregation refinement module (SARM), which mainly consists of a spatial-aware aggregation sub-module and a spatial-aware refinement sub-module to explore the spatial context of samples at the frame level. Then, we design a temporal-channel enhancement module (TCEM), which can capture the temporal-aware and channel-aware features of samples with the elaborately designed temporal-aware enhancement sub-module and channel-aware enhancement sub-module. Third, we introduce a cross-video relation module (CVRM), which can explore the relations across videos by utilizing the self-attention mechanism. Moreover, we design a prototype-centered mean absolute error loss to improve the feature learning capability of the proposed MRLN. Extensive experiments on four prevalent few-shot action recognition benchmarks show that the proposed MRLN can significantly outperform a variety of state-of-the-art few-shot action recognition methods. Especially, on the 5-way 1-shot setting, our MRLN respectively achieves 75.7%, 86.9%, 65.5% and 45.9% on the Kinetics, UCF101, HMDB51 and SSv2 datasets.
更多查看译文
AI 理解论文
溯源树
样例
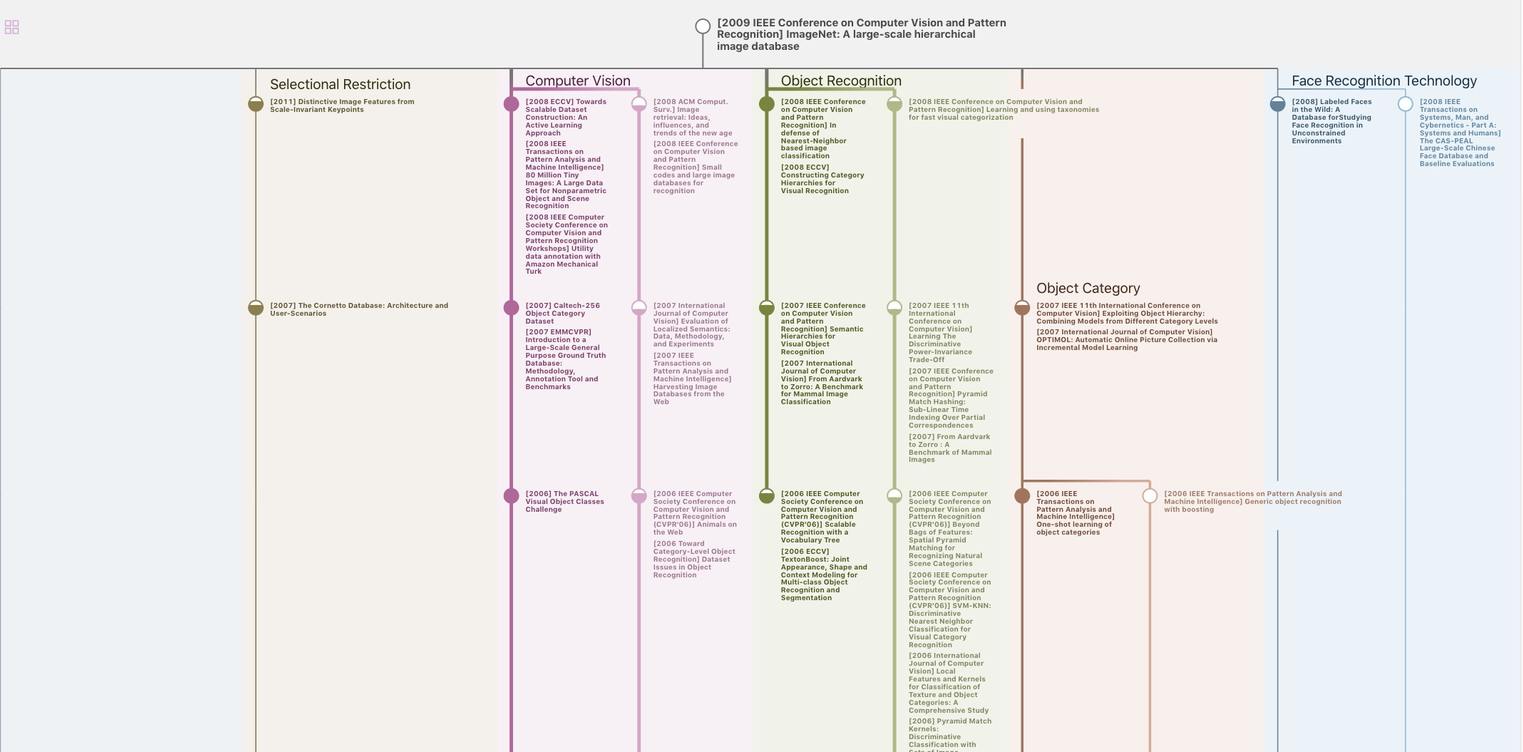
生成溯源树,研究论文发展脉络
Chat Paper
正在生成论文摘要