SSAE-AM: A Prediction Model for Fatigue Crack Growth
IEEE Internet of Things Journal(2024)
摘要
Real-time monitoring and prediction of damages form the basis of Artificial Intelligence for IT Operations (AIOps) in mechanical equipment, relying on the Internet of Things (IoT). Acoustic emission technology is widely used in Prognostic and Health Management (PHM) to monitor the growth of fatigue cracks online. Extracting and selecting high-quality acoustic emission features are crucial to the accuracy of fatigue crack prediction, as it helps establish the relationship between these features and fatigue crack growth (FCG). However, traditional artificially selected acoustic emission features are seriously affected by the signal amplitude threshold. To solve the above problems, we proposed a fatigue crack prediction model based on the improved stacked autoencoder and attention mechanism (SSAE-AM). The model can adaptively extract acoustic emission features that are strongly correlated with FCG by adding a supervision module to the stacked autoencoder (SAE) and using the attention mechanism (AM)to weight the fusion features. On this basis, the relationship model between acoustic emission features and FCG is established for crack prediction. Finally, we verify the validity of the model through experiments that monitor fatigue crack growth under different loading stresses. Compared with models that use other acoustic emission statistical features for crack prediction, the model proposed in this paper can achieve better prediction accuracy.
更多查看译文
关键词
Fatigue Crack Growth,Crack Predict,Acoustic Emission,Stacked Autoencoder,Attention Mechanism
AI 理解论文
溯源树
样例
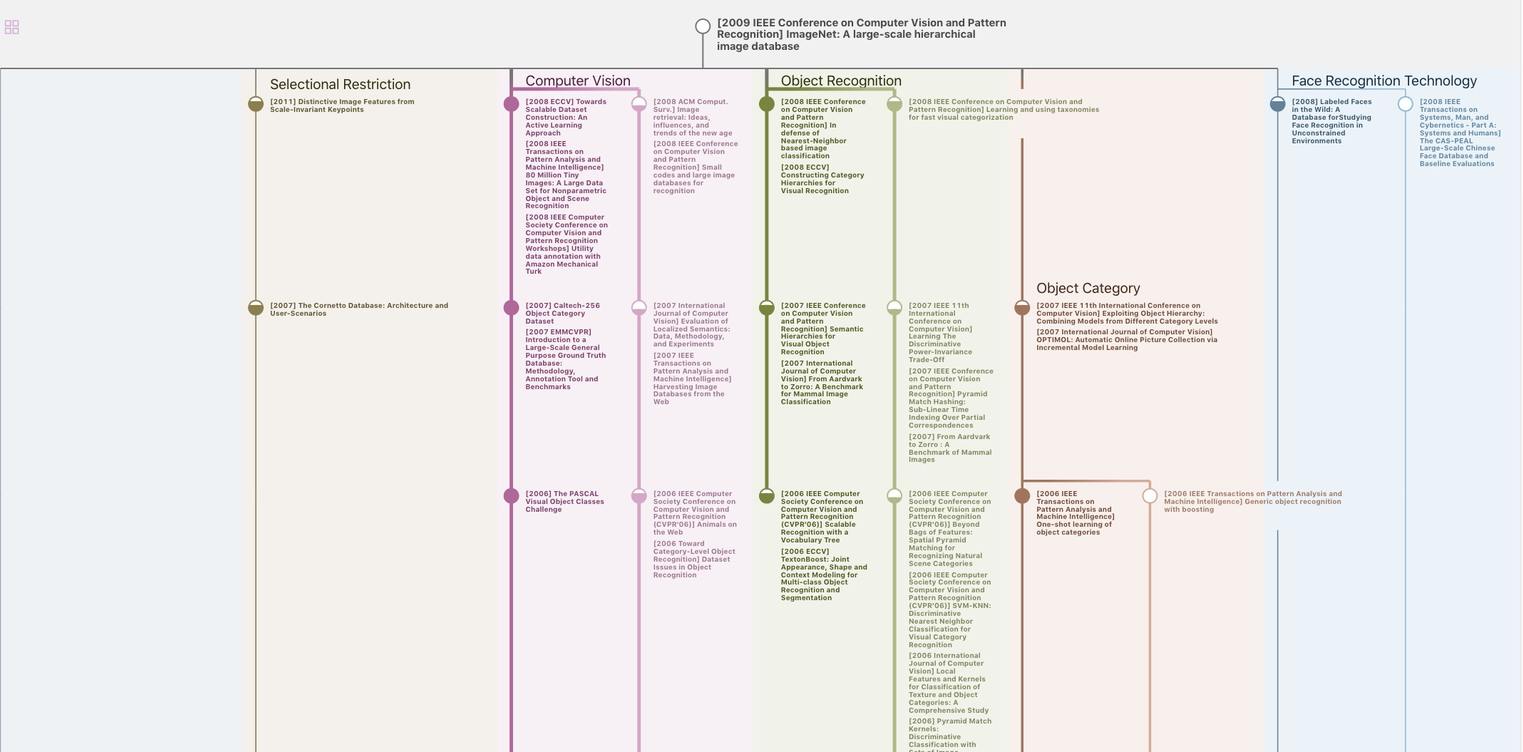
生成溯源树,研究论文发展脉络
Chat Paper
正在生成论文摘要