A Novel Cross-Domain Data Augmentation and Bearing Fault Diagnosis Method Based on an Enhanced Generative Model
IEEE Transactions on Instrumentation and Measurement(2024)
摘要
In actual industrial production, differences in production conditions lead to variations in the collected data distribution. This gives rise to a particular problem: while one set of conditions has complete status data available, another set only possesses data from the healthy state. Differences in data conditions result in limitations for diagnosing the new condition. To address this challenge, a method based on envelope order spectra for data generation is proposed. Initially, envelope and order analysis is conducted on raw vibration data to align envelope spectra across different domains and extract domain-independent signal components—the envelope order spectra. Subsequently, an enhanced Variational Autoencoder Generative Adversarial Network (VAEGAN) is trained using the envelope order spectra. The trained model is then employed to generate synthetic envelope order spectra, serving as data augmentation for another set of working conditions, thereby achieving cross-domain data augmentation. Next, the augmented envelope order spectra data is used to train a generic model for fault classification, enabling cross-domain fault diagnosis. Finally, the proposed approach is validated by testing it with real envelope order spectra data from a different working condition. Experimental results demonstrate that the proposed method can generate reliable fake data under diverse working conditions, accomplishing cross-domain data augmentation and fault diagnosis while preserving data privacy.
更多查看译文
关键词
envelope order spectrum,variational autoencoder generative adversarial networks,data imbalance,cross-domain data augmentation,fault diagnosis
AI 理解论文
溯源树
样例
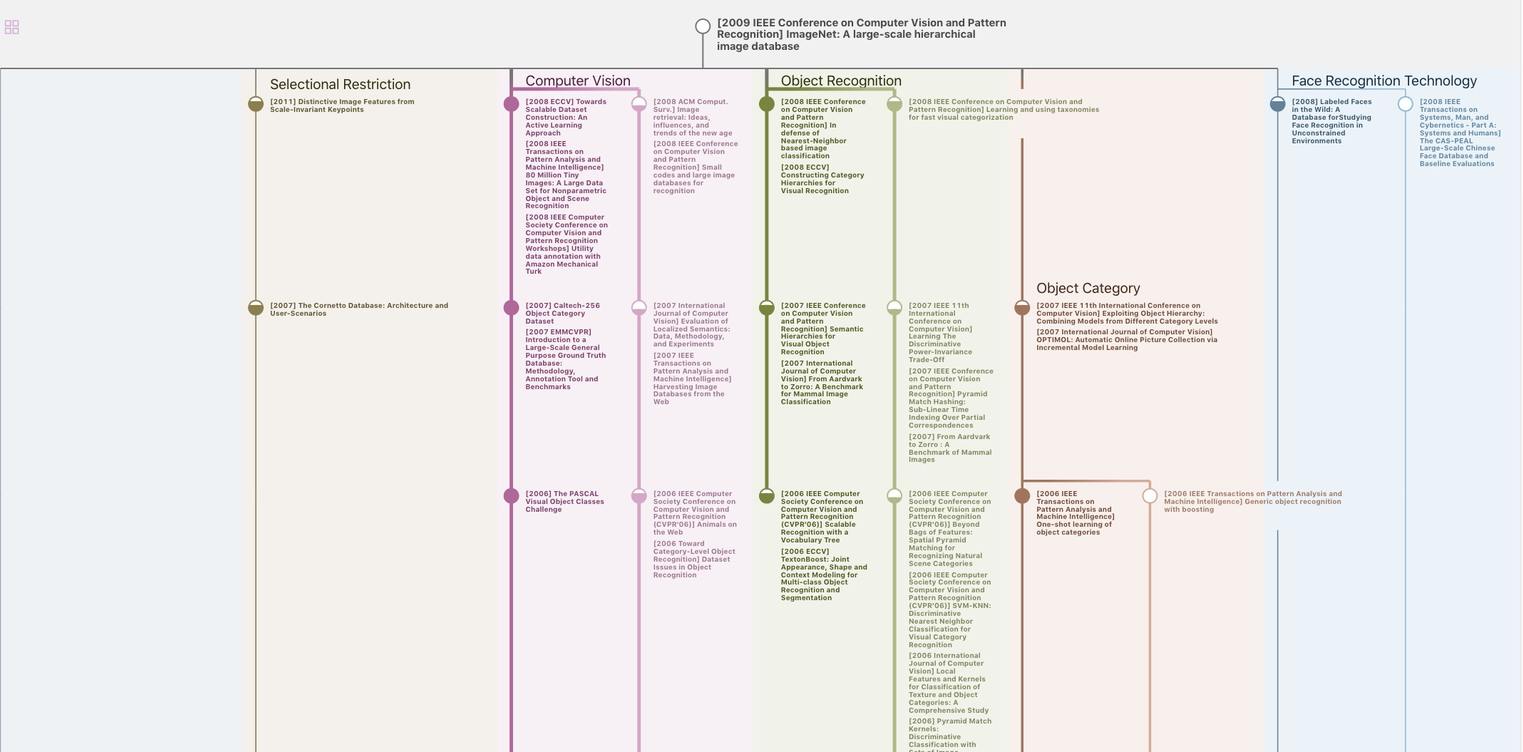
生成溯源树,研究论文发展脉络
Chat Paper
正在生成论文摘要