A Bilevel Periodically Interactive Evolutionary Algorithm for Personalized Service Customization in Wireless-Powered Cooperative MEC
IEEE Transactions on Emerging Topics in Computational Intelligence(2024)
Abstract
This article addresses the pricing scheme in a wireless-powered cooperative mobile edge computing (WP-CoMEC) system, focusing on personalized service customization. Traditional pricing schemes in such systems often assume a passive mode, with the service provider leading, and the device owner following. However, with the rise of personalized requirements, this paper proposes a novel approach where the device owner becomes an active participant in the pricing scheme, leading to personally customized services. The proposed pricing model formulates a bilevel multi-objective optimization problem, considering task offloading, resource allocation, and energy harvesting. This comprehensive approach ensures a more holistic optimization process. To address the computational challenges posed by the bilevel pricing model, this article proposes a bilevel periodically interactive evolutionary algorithm (BL-PIEA), which efficiently handles mixed variables, complex objective conflicts, and the inner nested structure of the bilevel pricing model. The proposed BL-PIEA is tested on ten instances, and the results indicate that BL-PIEA can effectively solve the proposed pricing model, showcasing superior performance in terms of reduced run time and saved evaluation budgets compared to other algorithms. With the proposed bilevel pricing model solved by BL-PIEA, the service provider can make out better pricing schemes that satisfy the device owner's requirements, so as to achieve a good personalized service customization.
MoreTranslated text
Key words
Bilevel optimization,mobile edge computing,personalized service customization,resource allocation,task offloading
求助PDF
上传PDF
View via Publisher
AI Read Science
AI Summary
AI Summary is the key point extracted automatically understanding the full text of the paper, including the background, methods, results, conclusions, icons and other key content, so that you can get the outline of the paper at a glance.
Example
Background
Key content
Introduction
Methods
Results
Related work
Fund
Key content
- Pretraining has recently greatly promoted the development of natural language processing (NLP)
- We show that M6 outperforms the baselines in multimodal downstream tasks, and the large M6 with 10 parameters can reach a better performance
- We propose a method called M6 that is able to process information of multiple modalities and perform both single-modal and cross-modal understanding and generation
- The model is scaled to large model with 10 billion parameters with sophisticated deployment, and the 10 -parameter M6-large is the largest pretrained model in Chinese
- Experimental results show that our proposed M6 outperforms the baseline in a number of downstream tasks concerning both single modality and multiple modalities We will continue the pretraining of extremely large models by increasing data to explore the limit of its performance
Upload PDF to Generate Summary
Must-Reading Tree
Example
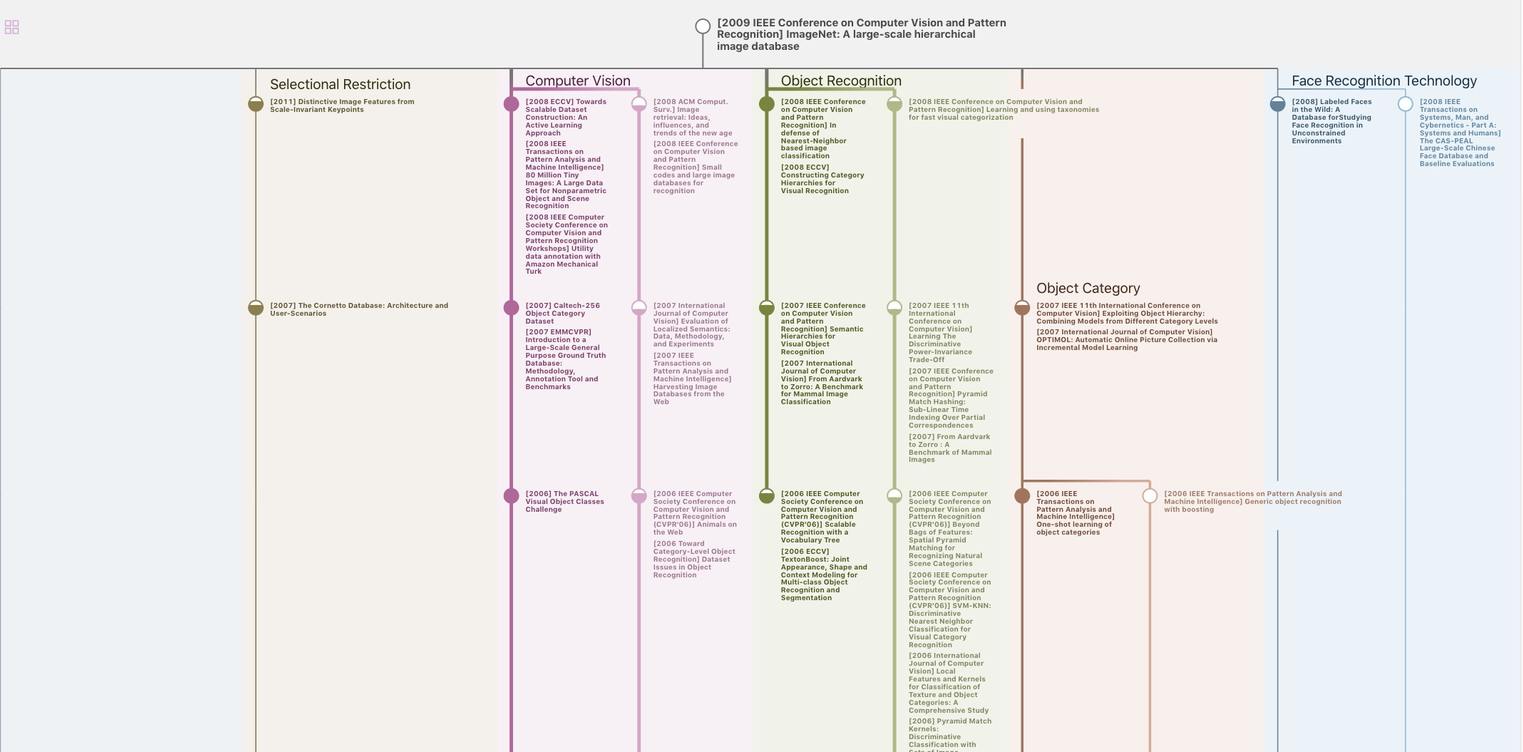
Generate MRT to find the research sequence of this paper
Related Papers
2008
被引用2771 | 浏览
2013
被引用722 | 浏览
2016
被引用23 | 浏览
2017
被引用304 | 浏览
2017
被引用695 | 浏览
2019
被引用149 | 浏览
2019
被引用73 | 浏览
JointDNN: an Efficient Training and Inference Engine for Intelligent Mobile Cloud Computing Services
2021
被引用283 | 浏览
2020
被引用200 | 浏览
2019
被引用247 | 浏览
2020
被引用27 | 浏览
2021
被引用326 | 浏览
2021
被引用9 | 浏览
2022
被引用229 | 浏览
2022
被引用16 | 浏览
2022
被引用3 | 浏览
2023
被引用31 | 浏览
2022
被引用3 | 浏览
2023
被引用5 | 浏览
Data Disclaimer
The page data are from open Internet sources, cooperative publishers and automatic analysis results through AI technology. We do not make any commitments and guarantees for the validity, accuracy, correctness, reliability, completeness and timeliness of the page data. If you have any questions, please contact us by email: report@aminer.cn
Chat Paper
GPU is busy, summary generation fails
Rerequest