Imitating via manipulability: Geometry-aware combined DMP with via-point and speed adaptation
Computers and Electrical Engineering(2024)
摘要
Manipulability ellipsoid on the Riemannian manifold provides an effective criterion for guiding the regulation of robot postures in an efficient and natural manner. While many manipulability-based learning by demonstration (LbD) methods achieve favorable performance in human-like manipulation, the adaptation of movement skills encapsulated in Symmetric Positive Definite (SPD) matrices and the implementation of manipulability-relevant skills related to velocity scaling are still largely open. In this paper, we develop a new framework based on Geometry-Aware Combined Dynamic Movement Primitives (GA-CDMP) for learning movement skills from demonstrations. The GA-CDMP model not only adapts learned trajectories to SPD-via-points but also establishes a correlation between SPD-based trajectories and position trajectories, enabling the adaptation of learned SPD-based trajectories across different non-linear motion velocity scales. The experimental evaluations show that our proposed method exhibits remarkable precision in passing through via-points positioned at a distance from the demonstration, while also substantially improving the invariant shape similarity in manipulability by approximately 65% compared to extended Kernelized Movement Primitives (KMPs). Moreover, our velocity adaptive approach achieves impressive success rates of up to 94% and demonstrates a notable reduction in execution time (11.72 ± 0.45 s), representing an almost 10% decrease in comparison to the state-of-the-art method employed in water-carrying tasks.
更多查看译文
关键词
Learning by demonstration,Manipulability ellipsoid,Robot movement skills,Geometry constraints,Velocity adaptation
AI 理解论文
溯源树
样例
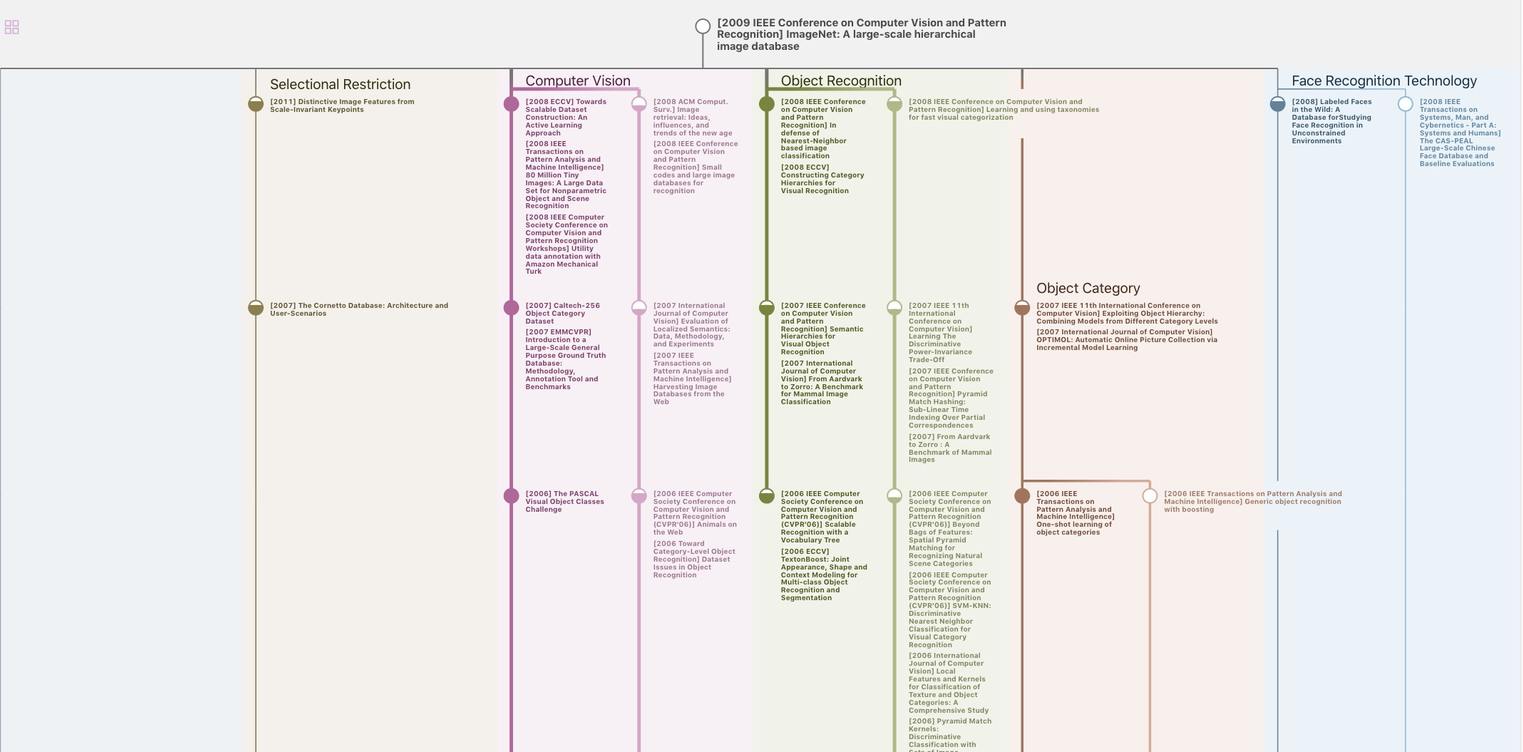
生成溯源树,研究论文发展脉络
Chat Paper
正在生成论文摘要