Improved MRI Brain Tumor Segmentation Method Based on UNet++
Academic Journal of Science and Technology(2024)
摘要
Brain tumors are one of the most common tumors with high mortality. Accurate detection and quantitative evaluation of brain tumors are necessary for patient treatment. A reliable and effective method for automatic segmentation of brain tumors by magnetic resonance imaging (MRI) plays a crucial role in patient treatment. In recent years, the Unet++ network structure has shown better performance in image segmentation. However, it is deficient in small-scale feature extraction and segmentation accuracy. In this study, the AC-ResUnet++ network was proposed to address the problems of Unet++ network mentioned above, which combined residual module, convolutional block attention module (CBAM) and Atrous spatial pyramidal pooling (ASPP). The residual module can solve the problem of gradient explosion in the deep network, CBAM can focus on local feature information and enhance the feature learning ability of the network for the target range, and ASPP module can generate different receptive fields and obtain multi-scale contextual feature information. The BraTS 2018 and BraTS 2019 datasets were used to test the proposed network. The Dice similarity coefficient of AC-ResUnet++ in whole tumor, tumor core and enhanced tumor are 0.8521, 0.8696 and 0.7868, respectively. Compared with Unet++ network, the Dice similarity coefficient increased by 1.22%, 5.08% and 2.03% on the baselines. The comprehensive results demonstrate that AC-ResUnet++ performs more effectively in improving the segmentation accuracy of brain tumor.
更多查看译文
AI 理解论文
溯源树
样例
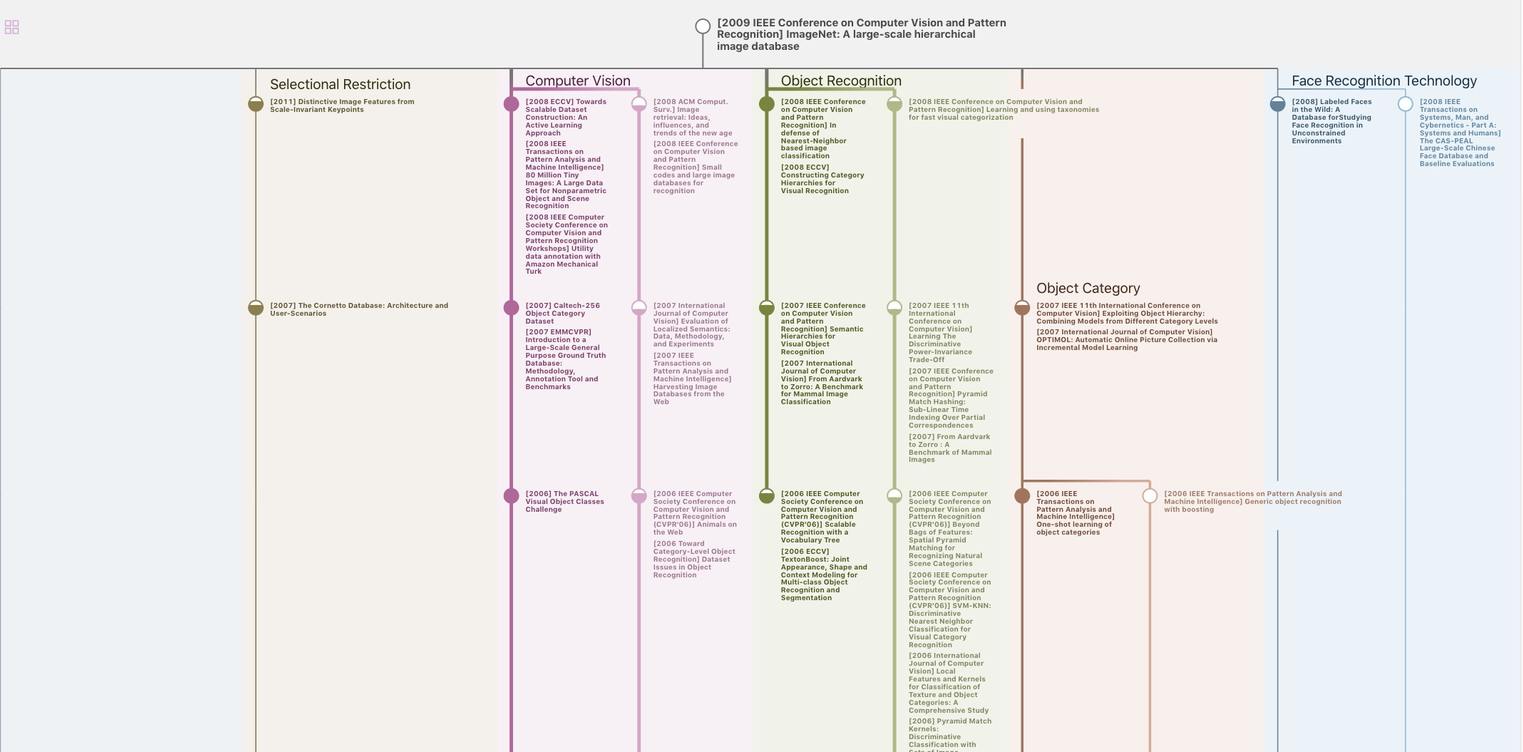
生成溯源树,研究论文发展脉络
Chat Paper
正在生成论文摘要