QTNet: Predicting Drug-Induced QT Prolongation With Artificial Intelligence–Enabled Electrocardiograms
JACC: Clinical Electrophysiology(2024)
摘要
Background
Prediction of drug-induced long QT syndrome (diLQTS) is of critical importance given its association with torsades de pointes. There is no reliable method for the outpatient prediction of diLQTS.
Objectives
This study sought to evaluate the use of a convolutional neural network (CNN) applied to electrocardiograms (ECGs) to predict diLQTS in an outpatient population.
Methods
We identified all adult outpatients newly prescribed a QT-prolonging medication between January 1, 2003, and March 31, 2022, who had a 12-lead sinus ECG in the preceding 6 months. Using risk factor data and the ECG signal as inputs, the CNN QTNet was implemented in TensorFlow to predict diLQTS.
Results
Models were evaluated in a held-out test dataset of 44,386 patients (57% female) with a median age of 62 years. Compared with 3 other models relying on risk factors or ECG signal or baseline QTc alone, QTNet achieved the best (P < 0.001) performance with a mean area under the curve of 0.802 (95% CI: 0.786-0.818). In a survival analysis, QTNet also had the highest inverse probability of censorship–weighted area under the receiver-operating characteristic curve at day 2 (0.875; 95% CI: 0.848-0.904) and up to 6 months. In a subgroup analysis, QTNet performed best among males and patients ≤50 years or with baseline QTc <450 ms. In an external validation cohort of solely suburban outpatient practices, QTNet similarly maintained the highest predictive performance.
Conclusions
An ECG-based CNN can accurately predict diLQTS in the outpatient setting while maintaining its predictive performance over time. In the outpatient setting, our model could identify higher-risk individuals who would benefit from closer monitoring.
更多查看译文
关键词
artificial intelligence,deep neural networks,drug-induced long QT syndrome,electrocardiogram deep learning,prolonged QT
AI 理解论文
溯源树
样例
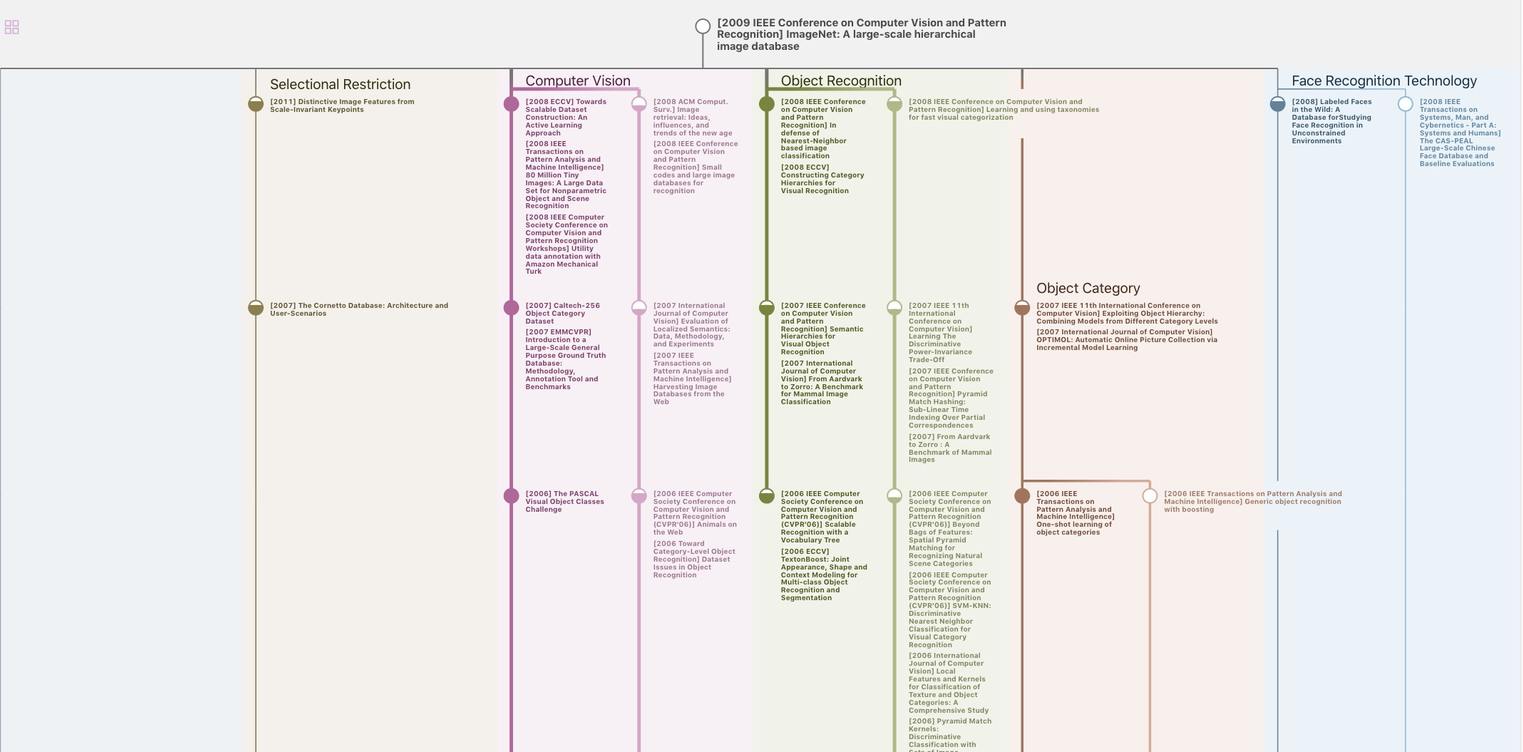
生成溯源树,研究论文发展脉络
Chat Paper
正在生成论文摘要