SCG-GFFE: A Self-Constructed graph fault feature extractor based on graph Auto-encoder algorithm for unlabeled single-variable vibration signals of harmonic reducer
Advanced Engineering Informatics(2024)
摘要
As a pivotal component in robotic systems, harmonic reducer fault diagnosis plays a crucial role in safe and stable operation; however, the lack of labelled fault samples hampers its effectiveness. This study introduces a self-constructed graph graph-autoencoder fault feature extractor (SCG-GFFE), a novel method that uses the Graph Auto-encoder (GAE) algorithm. SCG-GFFE leverages Graph Neural Networks (GNNs) for the unsupervised extraction of fault features, enhancing fault diagnosis in scenarios with limited labelled data. This approach overcomes the challenges of graph construction for fault diagnosis, particularly for single-sensor vibration signals. First, we developed the SCG-GFFE method for efficient fault feature extraction, which does not require complex domain expertise and is capable of generating graph structures. Second, we designed a suitably structured subgraph for dealing with single, unlabelled, non-multisource vibration signals. Third, a real-world harmonic reducer’s vibrational signal is utilised to build an experimental study and demonstrate the effectiveness of the SCG-GFFE method. The results of SCG-GFFE demonstrate remarkable accuracy in fault diagnosis, consistently exceeding 98% across various classifiers, and indicate that the proposed method can solve the problem of limited labelled data in harmonic reducer diagnosis.
更多查看译文
关键词
Self-constructed graph,Graph autoencoder,Single-variable vibration signals,Unsupervised fault feature extractor
AI 理解论文
溯源树
样例
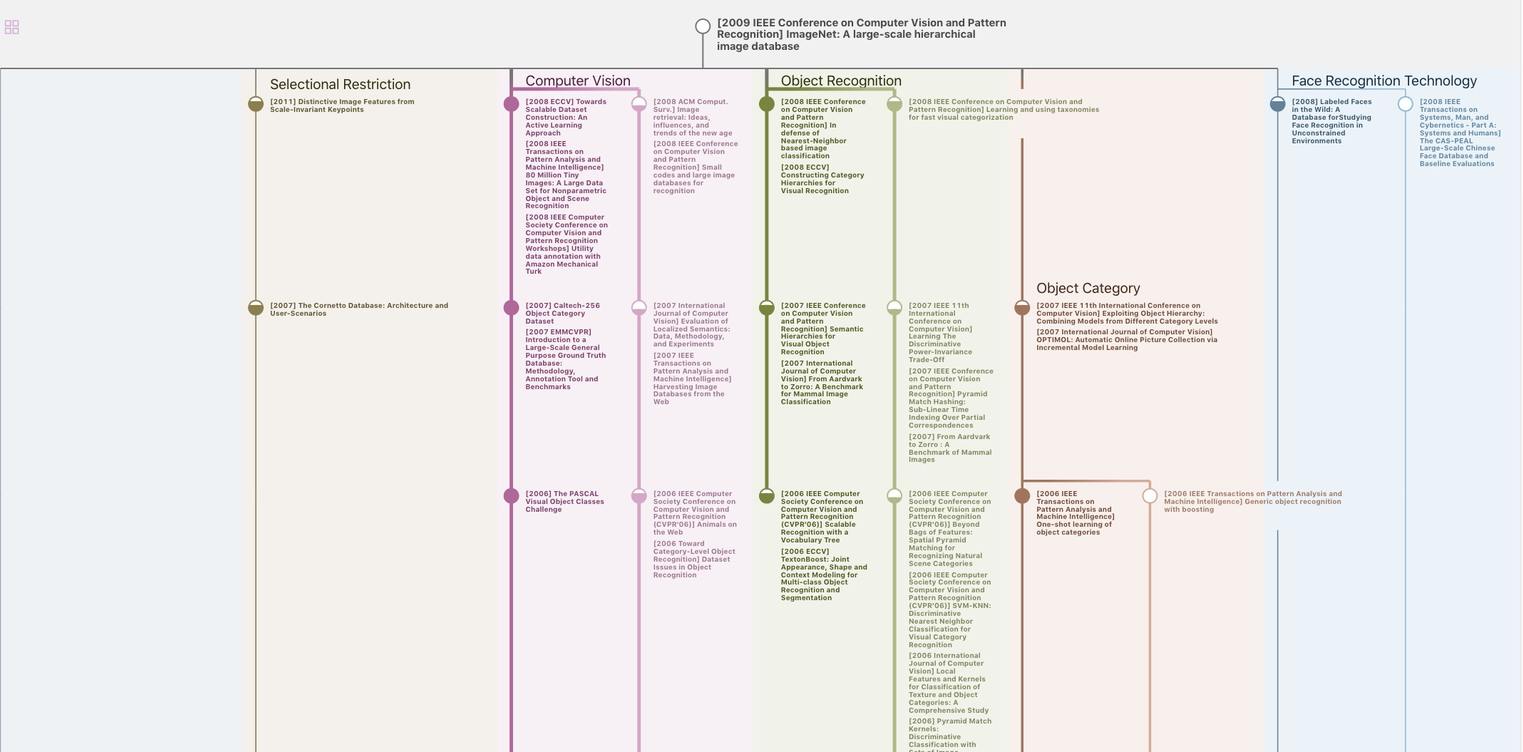
生成溯源树,研究论文发展脉络
Chat Paper
正在生成论文摘要