Performance Evaluation of Optical Transmission Based on Link Estimation by Using Deep Learning Techniques.
IEEE Access(2024)
摘要
In optical communication systems, the Q-factor is an important performance metric to evaluate the performance of an optical link. In this paper, a deep learning-based eye diagram analyzer is proposed to estimate the Q-factor. CNN architectures: LeNet, Wide ResNet, and Inception-v4 are used for ON-Off-Keying (OOK) and Pulse Amplitude Modulation (PAM) formats’ eye diagrams. The performance of these architectures is evaluated in terms of accuracy, Mean Squared Error (MSE), and error tolerance. This work shows that Wide ResNet demonstrates better performance in both OOK and PAM4 transmission schemes, achieving MSE values of 0.00188 and 0.00036, respectively. Additionally, it attains a high R-squared (R2) value of 0.9998. This deep learning-based eye diagram analyzer may be a promising approach for analyzing and optimizing optical communication systems without extensive human intervention.
更多查看译文
关键词
Deep neural network,Convolutional neural networks,Pulse amplitude modulation,Eye diagram,Non-return-to-zero,Q-factor,Intensity modulation–direct detection
AI 理解论文
溯源树
样例
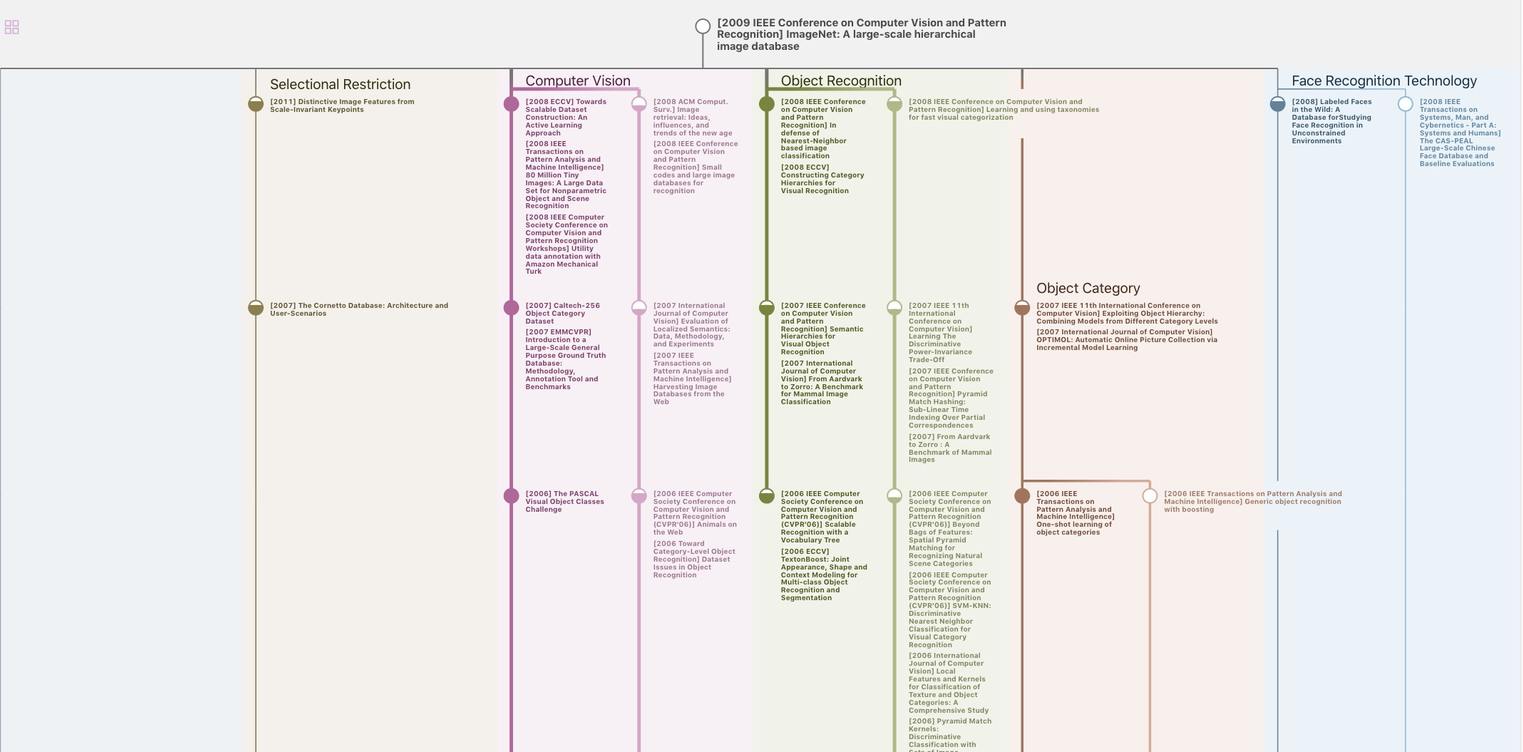
生成溯源树,研究论文发展脉络
Chat Paper
正在生成论文摘要