Initialization Seeds Facilitating Neural Network Quantization.
Data Compression Conference(2024)
摘要
Network quantization is promising to alleviate the storage and deployment burden for deep neural networks. However, existing methods focus on directly quantizing the network parameters but ignore the correlations between the initialized parameters (generated by random seeds) and the optimized parameters (obtained when the network training converges). In this paper, we reveal the redundancy between the initialized and optimized parameters and propose a novel quantization scheme for network parameters with rescaled initialization. We develop a novel quantization scheme for quantizing the delta parameters, i.e. , the difference between the optimized parameters and the rescaled initialization, as shown in Figure 1 . The scale is learned to adapt the weight regularization in training by minimizing the rounding error and penalizing the long-tailed distribution of the delta parameter. Adaptive rounding is further presented to calibrate the rounding direction of the delta parameter via a learnable bias. The proposed method can achieve high-efficiency prediction of network parameters and consistent performance gains with a trivial overhead of single random seed. Extensive experimental results demonstrate its superior performance on various popular network architectures, as exemplified in Table 1 .
更多查看译文
关键词
Network Quantization,Cylindrical,Network Parameters,Learning Bias
AI 理解论文
溯源树
样例
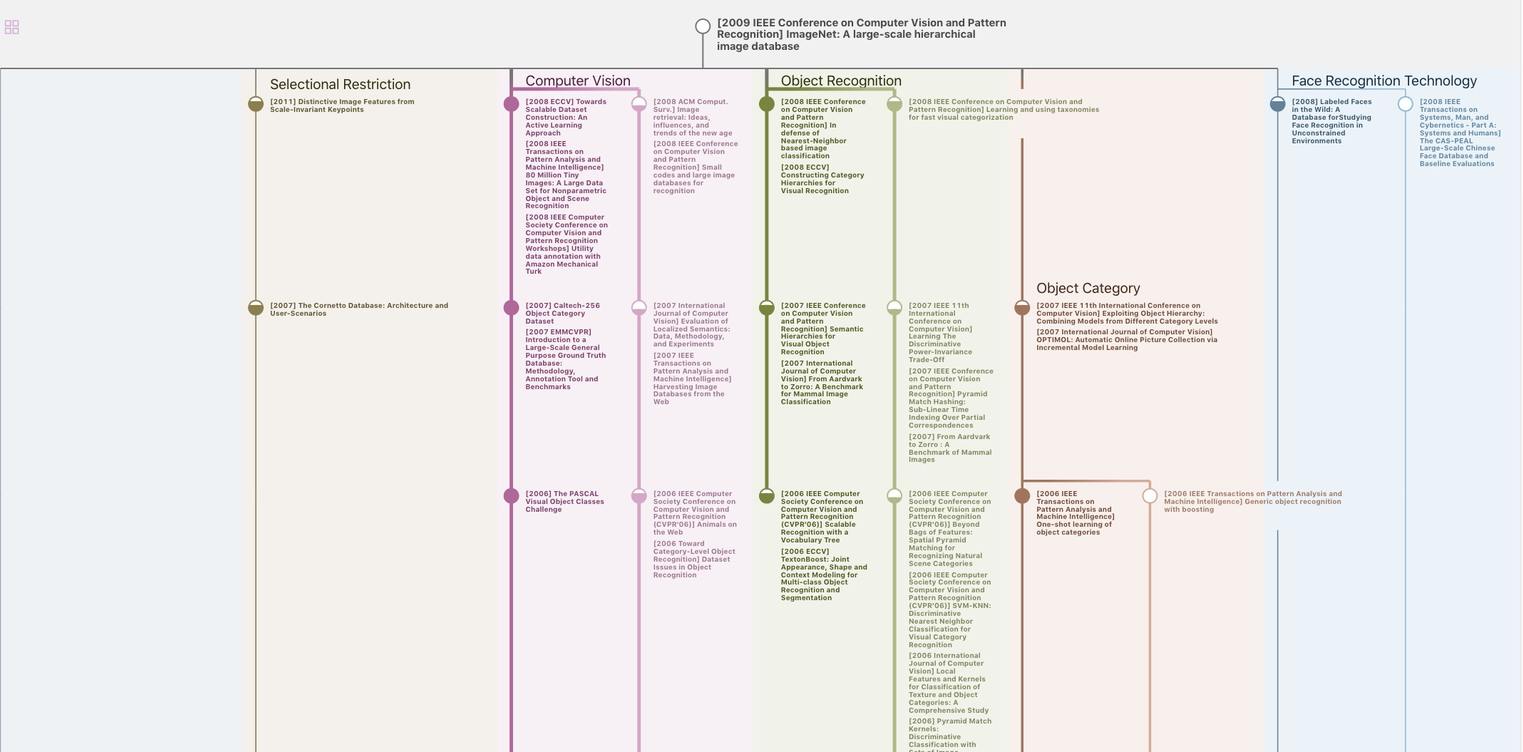
生成溯源树,研究论文发展脉络
Chat Paper
正在生成论文摘要