Bayesian spatial modelling for high dimensional seismic inverse problems
JOURNAL OF THE ROYAL STATISTICAL SOCIETY SERIES C-APPLIED STATISTICS(2016)
摘要
We study the application of Bayesian spatial modelling to seismic tomography, a geophysical, high dimensional, linearized inverse problem that infers the three-dimensional structure of the Earth's interior. We develop a spatial dependence model of seismic wave velocity variations in the Earth's mantle based on a Gaussian Matern field approximation. Using the theory of stochastic partial differential equations, this model quantifies the uncertainties in the parameter space by means of the integrated nested Laplace approximation. In resolution tests using simulated data and in inversions using real data, our model matches the performance of conventional deterministic optimization approaches in retrieving three-dimensional structure of the Earth's mantle. In addition it delivers estimates of the full parameter covariance matrix. Our model substantially improves on previous work relying on Markov chain Monte Carlo methods in terms of statistical misfits and computing time.
更多查看译文
关键词
High dimensional inverse problems,Integrated nested Laplace approximation,Seismic tomography,Spatial modelling on three-dimensional manifolds,Stochastic partial differential equations
AI 理解论文
溯源树
样例
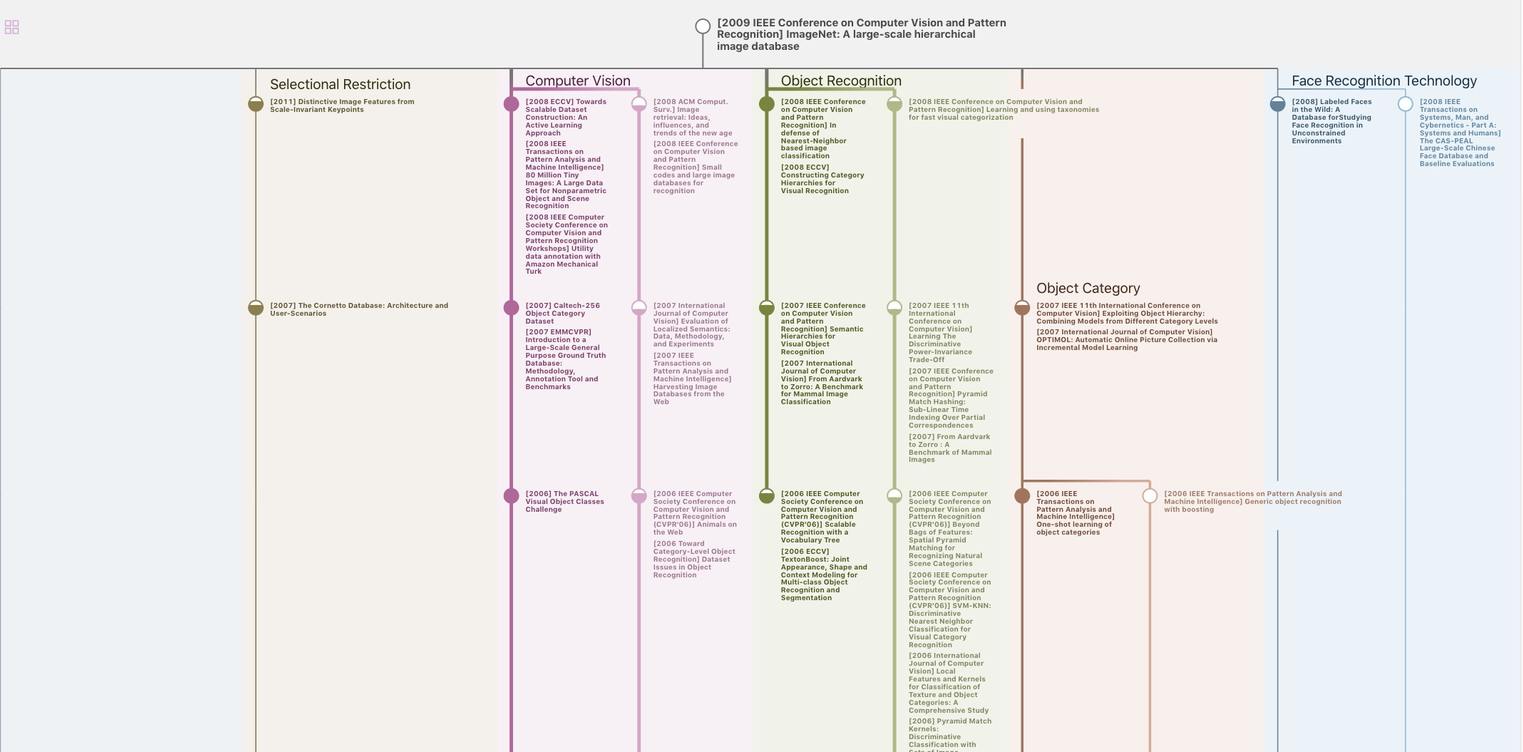
生成溯源树,研究论文发展脉络
Chat Paper
正在生成论文摘要