Towards Material Classification Of Scenes Using Active Thermography
2018 IEEE/RSJ INTERNATIONAL CONFERENCE ON INTELLIGENT ROBOTS AND SYSTEMS (IROS)(2018)
摘要
By briefly heating the local environment with a heat lamp and observing what happens with a thermal camera, robots could potentially infer properties of their surroundings. However, this form of active thermography introduces large signal variations compared to traditional active thermography, which has typically been used to characterize small regions of materials in carefully controlled settings. We demonstrate that a data-driven approach with modern machine learning methods can be used to classify material samples over relatively large surface areas and variable distances. We also introduce the use of z-normalization to improve material classification and reduce variation due to distance and heating intensity. Our best performing algorithm achieved an overall accuracy of 77.7% for multi-class classification among 12 materials placed at varying distances (20 cm, 30 cm, and 40 cm). The observations were made for 5 seconds with 1s of heating and 4s of cooling. We also provide a demonstration of performance with a multi-material scene.
更多查看译文
关键词
multimaterial scene,varying distances,multiclass classification,heating intensity,material classification,variable distances,relatively large surface areas,modern machine learning methods,data-driven approach,signal variations,thermal camera,heat lamp,size 20.0 cm,size 40.0 cm,size 30.0 cm,time 4.0 s,time 5.0 s,time 1.0 s
AI 理解论文
溯源树
样例
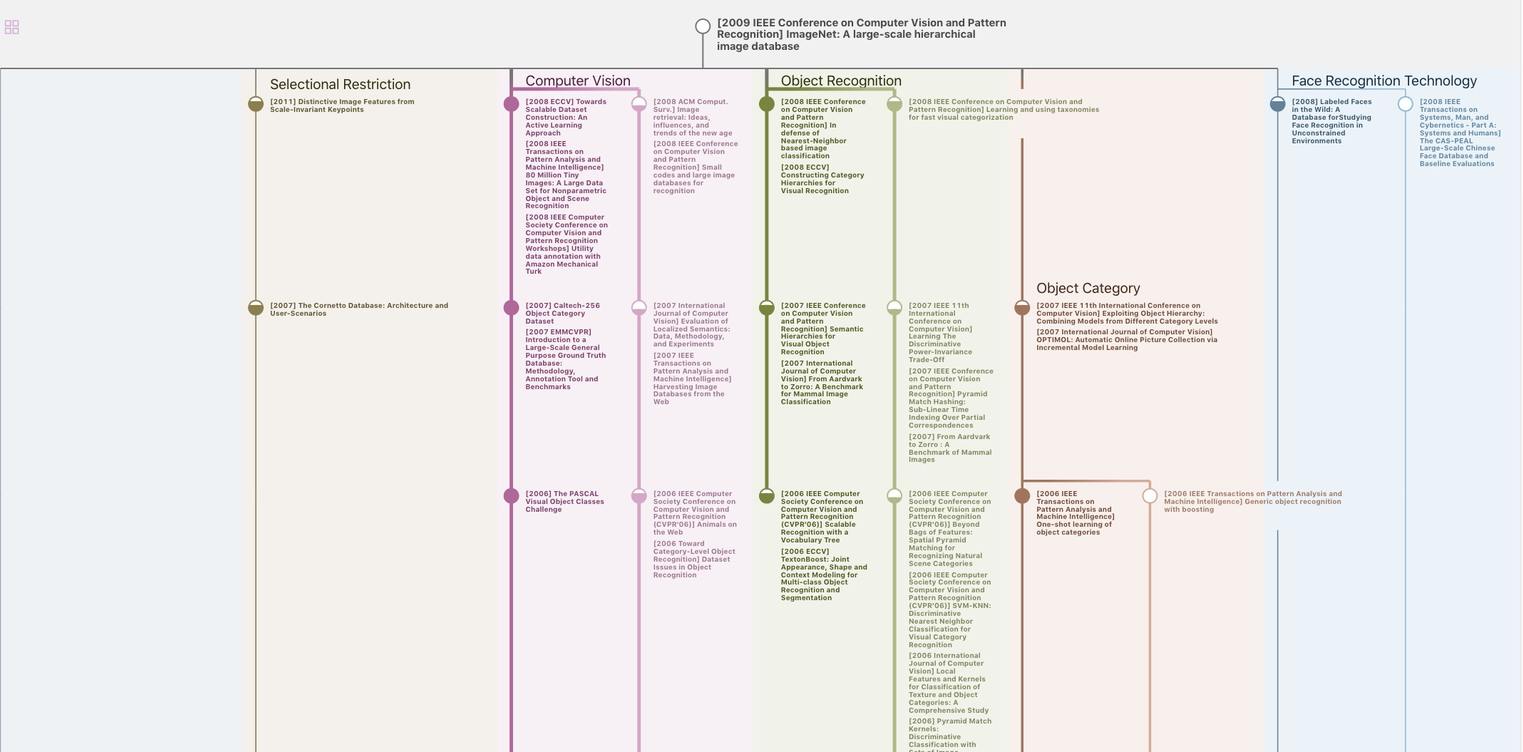
生成溯源树,研究论文发展脉络
Chat Paper
正在生成论文摘要