A Cascaded Deep Learning–Based Artificial Intelligence Algorithm for Automated Lesion Detection and Classification on Biparametric Prostate Magnetic Resonance Imaging
Academic Radiology(2022)
摘要
Rationale and objectives: Prostate MRI improves detection of clinically significant prostate cancer; however, its diagnostic performance has wide variation. Artificial intelligence (AI) has the potential to assist radiologists in the detection and classification of prostatic lesions. Herein, we aimed to develop and test a cascaded deep learning detection and classification system trained on biparametric prostate MRI using PI-RADS for assisting radiologists during prostate MRI read out. Materials and Methods: T2-weighted, diffusion-weighted (ADC maps, high b value DWI) MRI scans obtained at 3 Tesla from two institu-tions (n = 1043 in-house and n = 347 Prostate-X, respectively) acquired between 2015 to 2019 were used for model training, validation, testing. All scans were retrospectively reevaluated by one radiologist. Suspicious lesions were contoured and assigned a PI-RADS cate-gory. A 3D U-Net-based deep neural network was used to train an algorithm for automated detection and segmentation of prostate MRI lesions. Two 3D residual neural network were used for a 4-class classification task to predict PI-RADS categories 2 to 5 and BPH. Training and validation used 89% (n = 1290 scans) of the data using 5 fold cross-validation, the remaining 11% (n = 150 scans) were used for inde-pendent testing. Algorithm performance at lesion level was assessed using sensitivities, positive predictive values (PPV), false discovery rates (FDR), classification accuracy, Dice similarity coefficient (DSC). Additional analysis was conducted to compare AI algorithm's lesion detection performance with targeted biopsy results. Results: Median age was 66 years (IQR = 60-71), PSA 6.7 ng/ml (IQR = 4.7-9.9) from in-house cohort. In the independent test set, algo-rithm correctly detected 111 of 198 lesions leading to 56.1% (49.3%-62.6%) sensitivity. PPV was 62.7% (95% CI 54.7%-70.7%) with FDR of 37.3% (95% CI 29.3%-45.3%). Of 79 true positive lesions, 82.3% were tumor positive at targeted biopsy, whereas of 57 false neg-ative lesions, 50.9% were benign at targeted biopsy. Median DSC for lesion segmentation was 0.359. Overall PI-RADS classification accuracy was 30.8% (95% CI 24.6%-37.8%). Conclusion: Our cascaded U-Net, residual network architecture can detect, classify cancer suspicious lesions at prostate MRI with good detection, reasonable classification performance metrics.
更多查看译文
关键词
Prostate cancer,biparametric,magnetic resonance imaging,detection,artificial intelligence
AI 理解论文
溯源树
样例
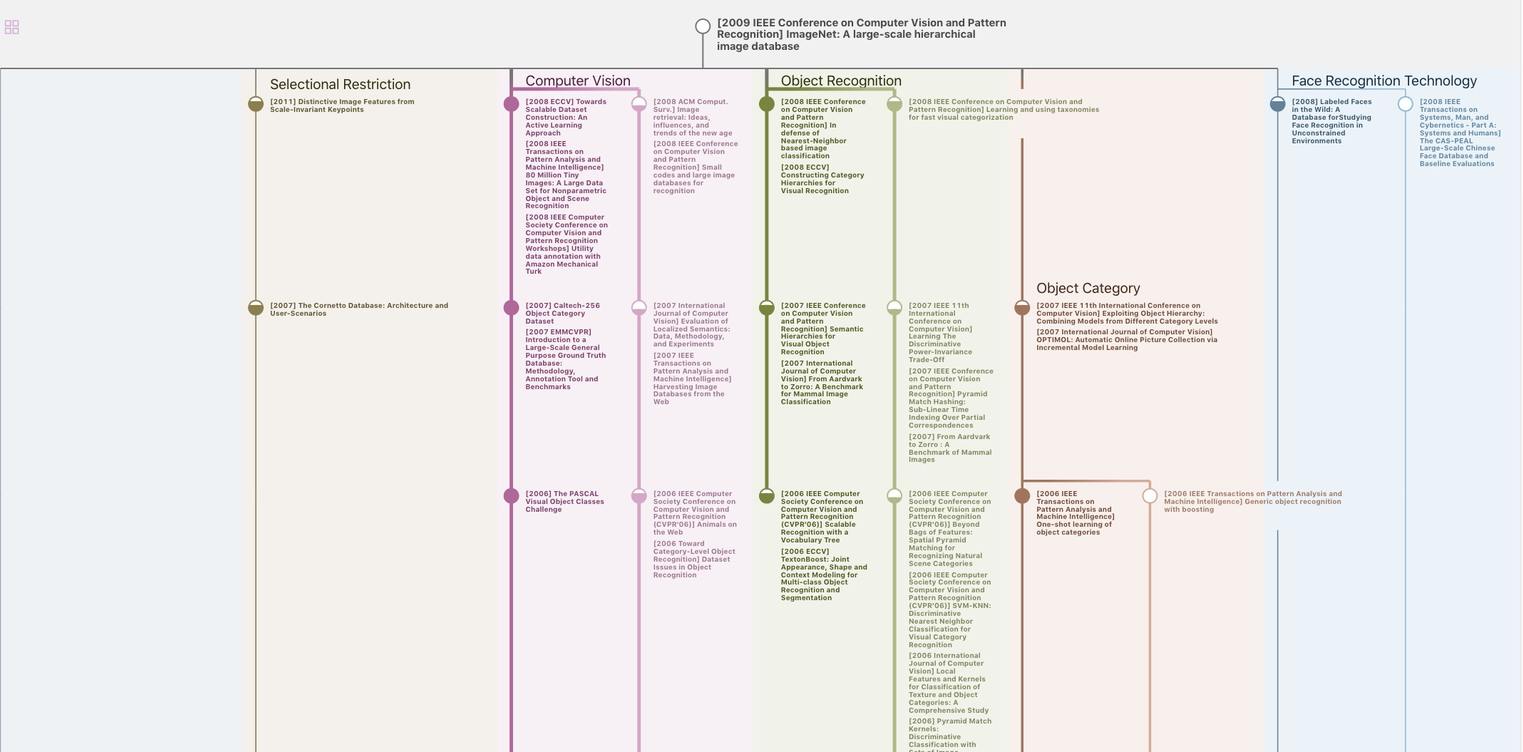
生成溯源树,研究论文发展脉络
Chat Paper
正在生成论文摘要