Learning Generalizable Vision-Tactile Robotic Grasping Strategy for Deformable Objects Via Transformer
IEEE-ASME TRANSACTIONS ON MECHATRONICS(2024)
关键词
Deep learning,perception for grasping and manipulation,visual and tactile sensing
AI 理解论文
溯源树
样例
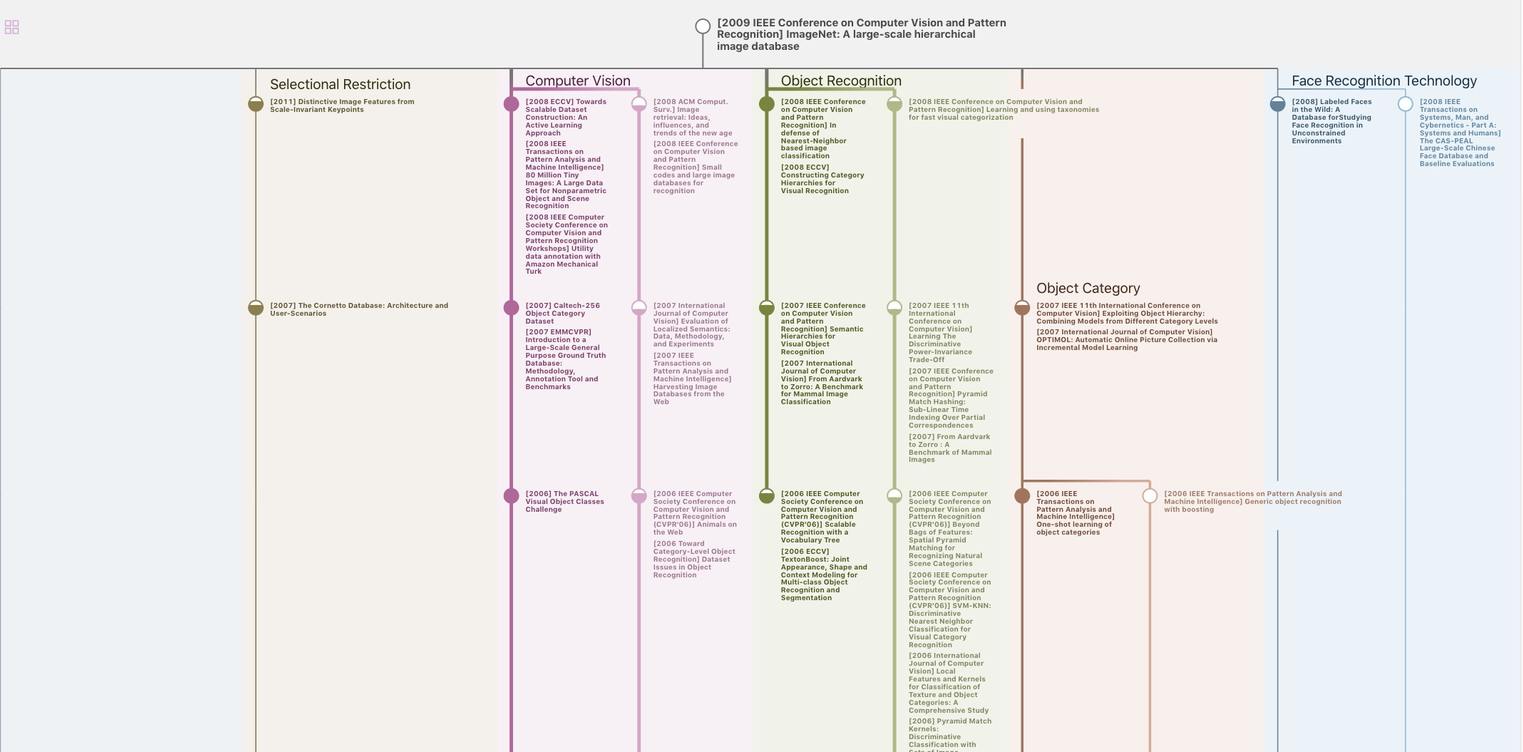
生成溯源树,研究论文发展脉络
Chat Paper
正在生成论文摘要