Fast Millimeter-Wave Base Station Discovery Via Data-driven Beam Training Optimization
International Symposium on Wireless Communication Systems (ISWCS)(2022)
School of Communication Engineering | College of Information Science and Electronic Engineering
Abstract
Millimeter-Wave (mm-wave) communications is an important element of 5G. Due to the high propagation loss of mm-wave signals, directional transmissions are required even in the initial access (IA), where the base station (BS) needs to broadcast the reference signals with beamforming to reach sufficient coverage ranges. Sequential scanning with narrow beams at the BS, without considering the non-uniform distribution of user equipment (UE) in the angular space, may lead to long IA delay at UEs. To reduce the IA delay, we propose a data-driven approach that learns the spatial distribution of UEs from the historical channels of UEs served by the BS and a beam identification method based on density-based spatial clustering of applications with noise (DBSCAN) to find the optimized set of beams to match to the distribution of the UEs. Two time resource allocation strategies are then investigated to evaluate the performance of IA based on the optimized beam set identified according to the UE distribution. Numerical results via realistic ray-tracing experiments demonstrate the performance improvement of the proposed approach over sequential beam training and omnidirectional training.
MoreTranslated text
Key words
Millimeter wave,beamforming,Initial Access (IA),DBSCAN
求助PDF
上传PDF
View via Publisher
AI Read Science
AI Summary
AI Summary is the key point extracted automatically understanding the full text of the paper, including the background, methods, results, conclusions, icons and other key content, so that you can get the outline of the paper at a glance.
Example
Background
Key content
Introduction
Methods
Results
Related work
Fund
Key content
- Pretraining has recently greatly promoted the development of natural language processing (NLP)
- We show that M6 outperforms the baselines in multimodal downstream tasks, and the large M6 with 10 parameters can reach a better performance
- We propose a method called M6 that is able to process information of multiple modalities and perform both single-modal and cross-modal understanding and generation
- The model is scaled to large model with 10 billion parameters with sophisticated deployment, and the 10 -parameter M6-large is the largest pretrained model in Chinese
- Experimental results show that our proposed M6 outperforms the baseline in a number of downstream tasks concerning both single modality and multiple modalities We will continue the pretraining of extremely large models by increasing data to explore the limit of its performance
Upload PDF to Generate Summary
Must-Reading Tree
Example
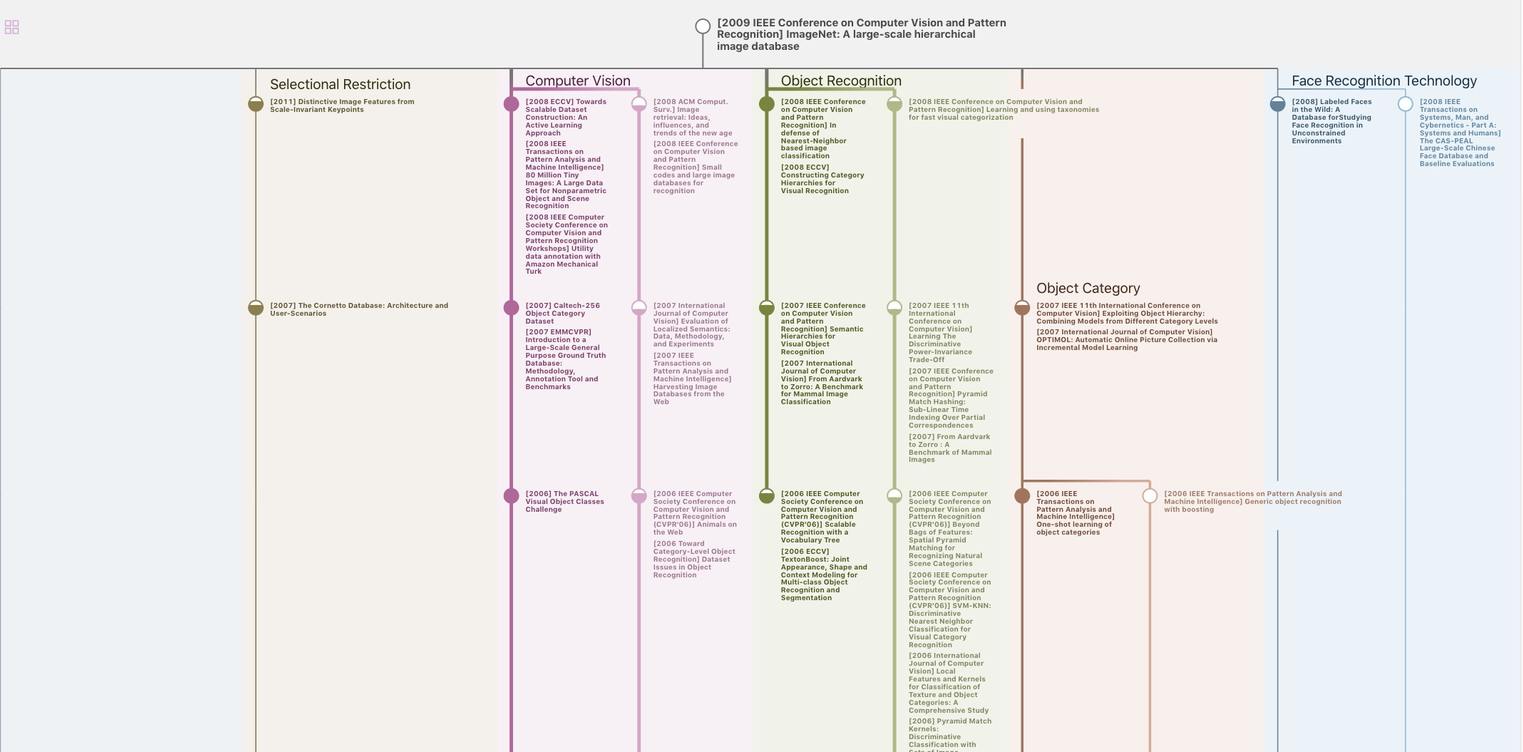
Generate MRT to find the research sequence of this paper
Related Papers
2012
被引用33 | 浏览
2017
被引用36 | 浏览
2018
被引用63 | 浏览
2020
被引用82 | 浏览
Data Disclaimer
The page data are from open Internet sources, cooperative publishers and automatic analysis results through AI technology. We do not make any commitments and guarantees for the validity, accuracy, correctness, reliability, completeness and timeliness of the page data. If you have any questions, please contact us by email: report@aminer.cn
Chat Paper
GPU is busy, summary generation fails
Rerequest