On Designing a Learning Robot: Improving Morphology for Enhanced Task Performance and Learning
2023 IEEE/RSJ INTERNATIONAL CONFERENCE ON INTELLIGENT ROBOTS AND SYSTEMS, IROS(2023)
Abstract
As robots become more prevalent, optimizing their design for better performance and efficiency is becoming increasingly important. However, current robot design practices overlook the impact of perception and design choices on a robot's learning capabilities. To address this gap, we propose a comprehensive methodology that accounts for the interplay between the robot's perception, hardware characteristics, and task requirements. Our approach optimizes the robot's morphology holistically, leading to improved learning and task execution proficiency. To achieve this, we introduce a Morphology-AGnostIc Controller (MAGIC), which helps with the rapid assessment of different robot designs. The MAGIC policy is efficiently trained through a novel PRIvileged Single-stage learning via latent alignMent (PRISM) framework, which also encourages behaviors that are typical of robot onboard observation. Our simulation-based results demonstrate that morphologies optimized holistically improve the robot performance by 15-20% on various manipulation tasks, and require 25x less data to match human-expert made morphology performance. In summary, our work contributes to the growing trend of learning-based approaches in robotics and emphasizes the potential in designing robots that facilitate better learning. The project's website can be found at learning-robot.github.io
MoreTranslated text
Key words
learning robot,morphology,designing
AI Read Science
Must-Reading Tree
Example
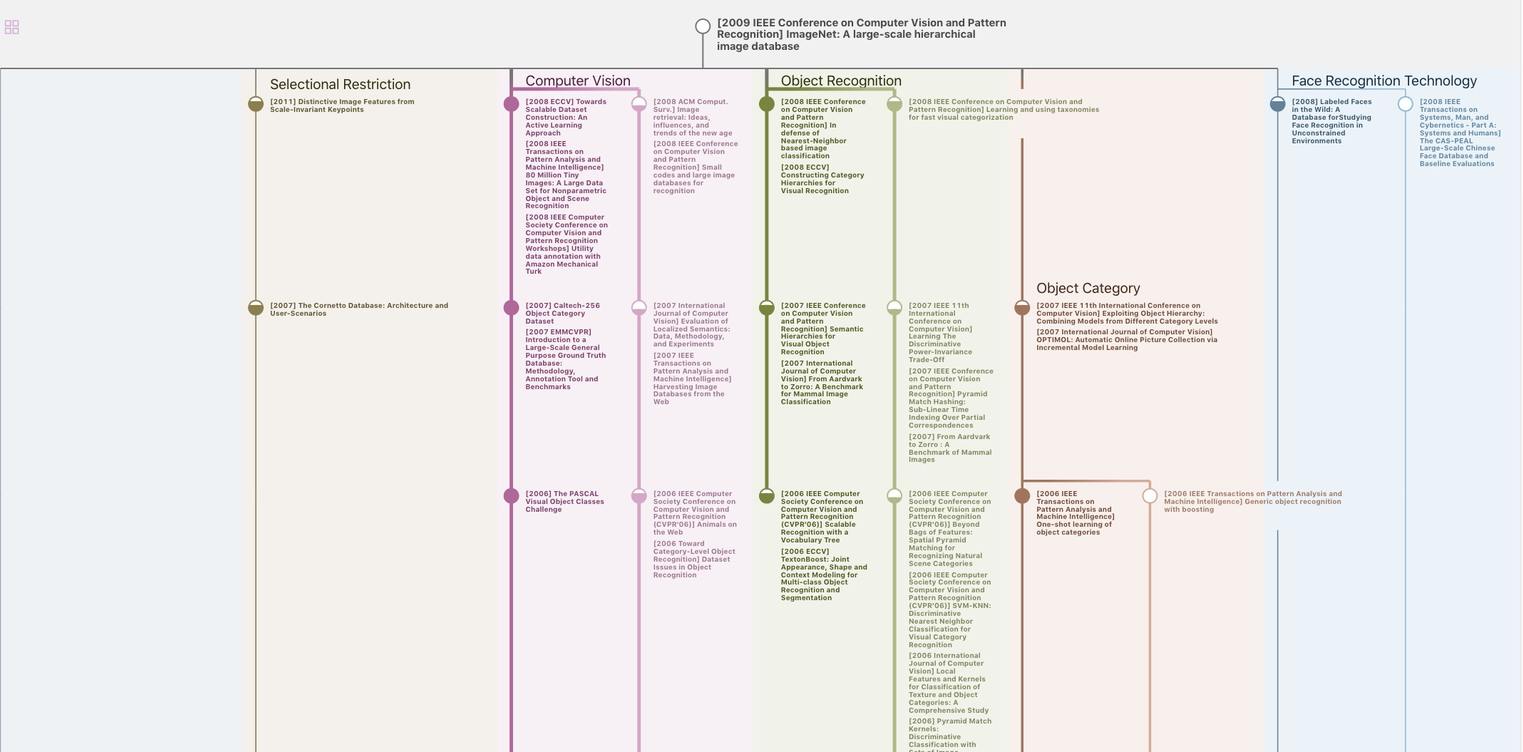
Generate MRT to find the research sequence of this paper
Chat Paper
Summary is being generated by the instructions you defined