A Digital LDO in 22-Nm CMOS with a 4-B Self-Triggered Binary Search Windowed Flash ADC Featuring Analog Layout Generator Framework
IEEE SOLID-STATE CIRCUITS LETTERS(2023)
Tsinghua Univ | Intel Labs
Abstract
This letter presents an analog layout generator-based digital LDO (DLDO) with a self-triggered binary search windowed flash analog-to-digital conversion (ADC) in 22-nm CMOS. A self-triggered binary search mechanism with a delay-based architecture is proposed to reduce the exponentially growing kickback noise and energy consumption of a traditional flash ADC down to the level of a SAR ADC while maintaining its high-speed feature. To conquer the complexity bottleneck of SoC development in FinFET technology, a practical analog layout generation framework is proposed to maximize the productivity of implementing analog circuit blocks in the scaled CMOS process. To meet the performance, area and reliability specifications across a variety of circuits, the methodology allows varying levels of constraints from designers, thus significantly improving the physical design time & effort up to $60\times $ compared with conventional manual approach. The DLDO features 3.55-ps FoM and fully automatic generation.
MoreTranslated text
Key words
Analog layout automation,analog-to-digital conversion (ADC),computer-aided design,low dropout regulator (LDO),power management
求助PDF
上传PDF
View via Publisher
AI Read Science
AI Summary
AI Summary is the key point extracted automatically understanding the full text of the paper, including the background, methods, results, conclusions, icons and other key content, so that you can get the outline of the paper at a glance.
Example
Background
Key content
Introduction
Methods
Results
Related work
Fund
Key content
- Pretraining has recently greatly promoted the development of natural language processing (NLP)
- We show that M6 outperforms the baselines in multimodal downstream tasks, and the large M6 with 10 parameters can reach a better performance
- We propose a method called M6 that is able to process information of multiple modalities and perform both single-modal and cross-modal understanding and generation
- The model is scaled to large model with 10 billion parameters with sophisticated deployment, and the 10 -parameter M6-large is the largest pretrained model in Chinese
- Experimental results show that our proposed M6 outperforms the baseline in a number of downstream tasks concerning both single modality and multiple modalities We will continue the pretraining of extremely large models by increasing data to explore the limit of its performance
Upload PDF to Generate Summary
Must-Reading Tree
Example
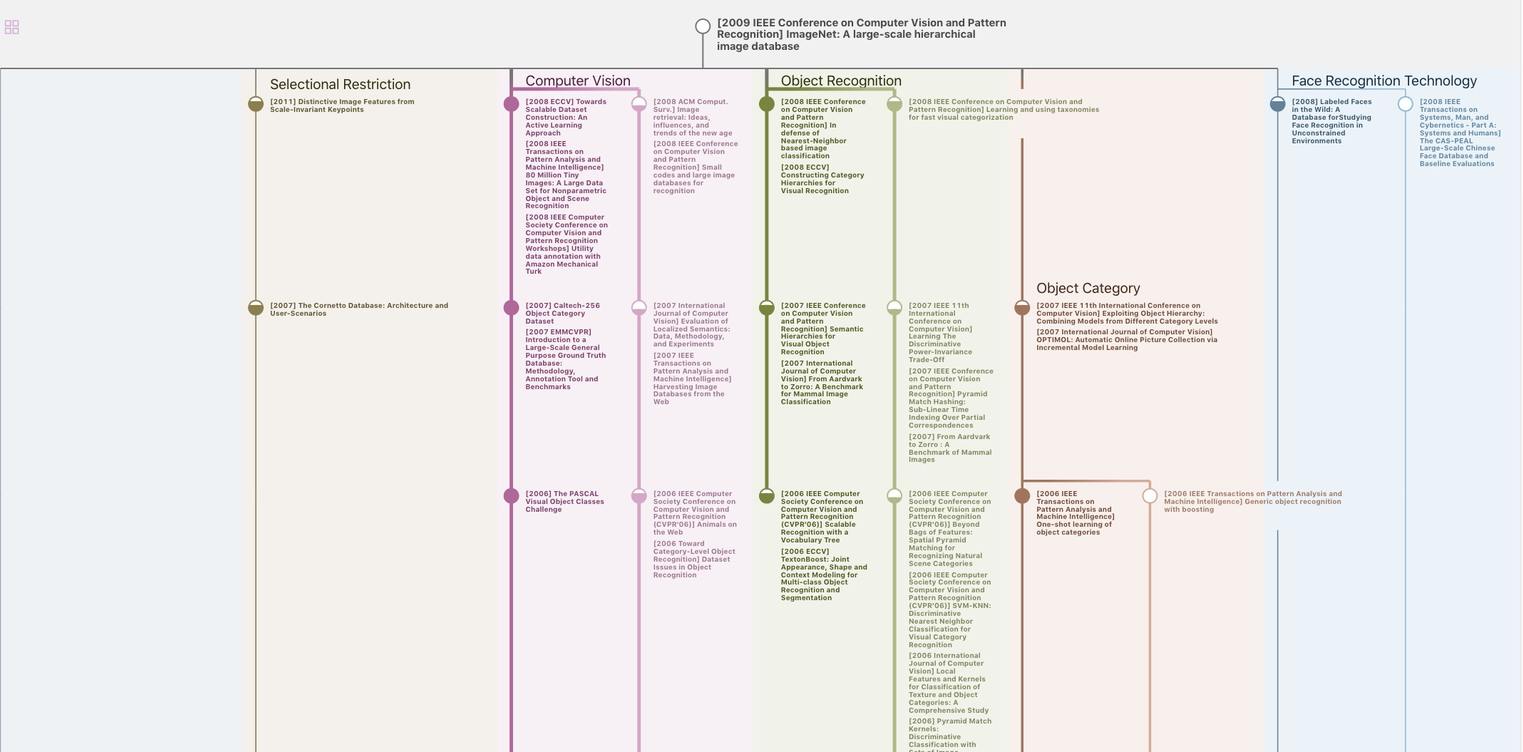
Generate MRT to find the research sequence of this paper
Related Papers
Data Disclaimer
The page data are from open Internet sources, cooperative publishers and automatic analysis results through AI technology. We do not make any commitments and guarantees for the validity, accuracy, correctness, reliability, completeness and timeliness of the page data. If you have any questions, please contact us by email: report@aminer.cn
Chat Paper