Progressive Bidirectional Feature Extraction and Enhancement Network for Quality Evaluation of Night-Time Images
IEEE TRANSACTIONS ON MULTIMEDIA(2024)
Ningbo Univ | Natl Astron Observ CAS | Gwangju Inst Sci & Technol GIST
Abstract
Blind image quality assessment (BIQA) has received increasing attention in the past decades. However, it still remains inadequately researched on BIQA for night-time images suffering from the diverse authentic degradations. Since the intrinsic content degradations of night-time images are highly related to the illumination, how to use the connection between content and illumination to enhance the feature representation ability is the key issue in designing BIQA methods for night-time images. In this article, we first construct an ultra-high-definition night-time image dataset (UHD-NID) with high image resolution and abundant parameter settings. UHD-NID contains 1600 images with a high resolution of 5616 x 3744, and each group of images contains ten exposure levels. Then, we conduct subjective assessment and analyze the subjective data to obtain a mean opinion score to each image in UHD-NID. To enhance the feature representation ability in content and illumination, we propose a Progressive Bidirectional Feature Extraction and Enhancement Network (PBFEE-Net). In addition, we use a decomposition network to decompose the input image into the reflectance and illumination, which can facilitate the ability of feature extraction to some extent. The experimental results show that our proposed method achieves superior performance in evaluating the quality of night-time images.
MoreTranslated text
Key words
Lighting,Feature extraction,Degradation,Image resolution,Reflectivity,Artificial neural networks,Visualization,Bidirectional enhancement,blind image quality assessment,image decomposition,night-time image
求助PDF
上传PDF
View via Publisher
AI Read Science
AI Summary
AI Summary is the key point extracted automatically understanding the full text of the paper, including the background, methods, results, conclusions, icons and other key content, so that you can get the outline of the paper at a glance.
Example
Background
Key content
Introduction
Methods
Results
Related work
Fund
Key content
- Pretraining has recently greatly promoted the development of natural language processing (NLP)
- We show that M6 outperforms the baselines in multimodal downstream tasks, and the large M6 with 10 parameters can reach a better performance
- We propose a method called M6 that is able to process information of multiple modalities and perform both single-modal and cross-modal understanding and generation
- The model is scaled to large model with 10 billion parameters with sophisticated deployment, and the 10 -parameter M6-large is the largest pretrained model in Chinese
- Experimental results show that our proposed M6 outperforms the baseline in a number of downstream tasks concerning both single modality and multiple modalities We will continue the pretraining of extremely large models by increasing data to explore the limit of its performance
Upload PDF to Generate Summary
Must-Reading Tree
Example
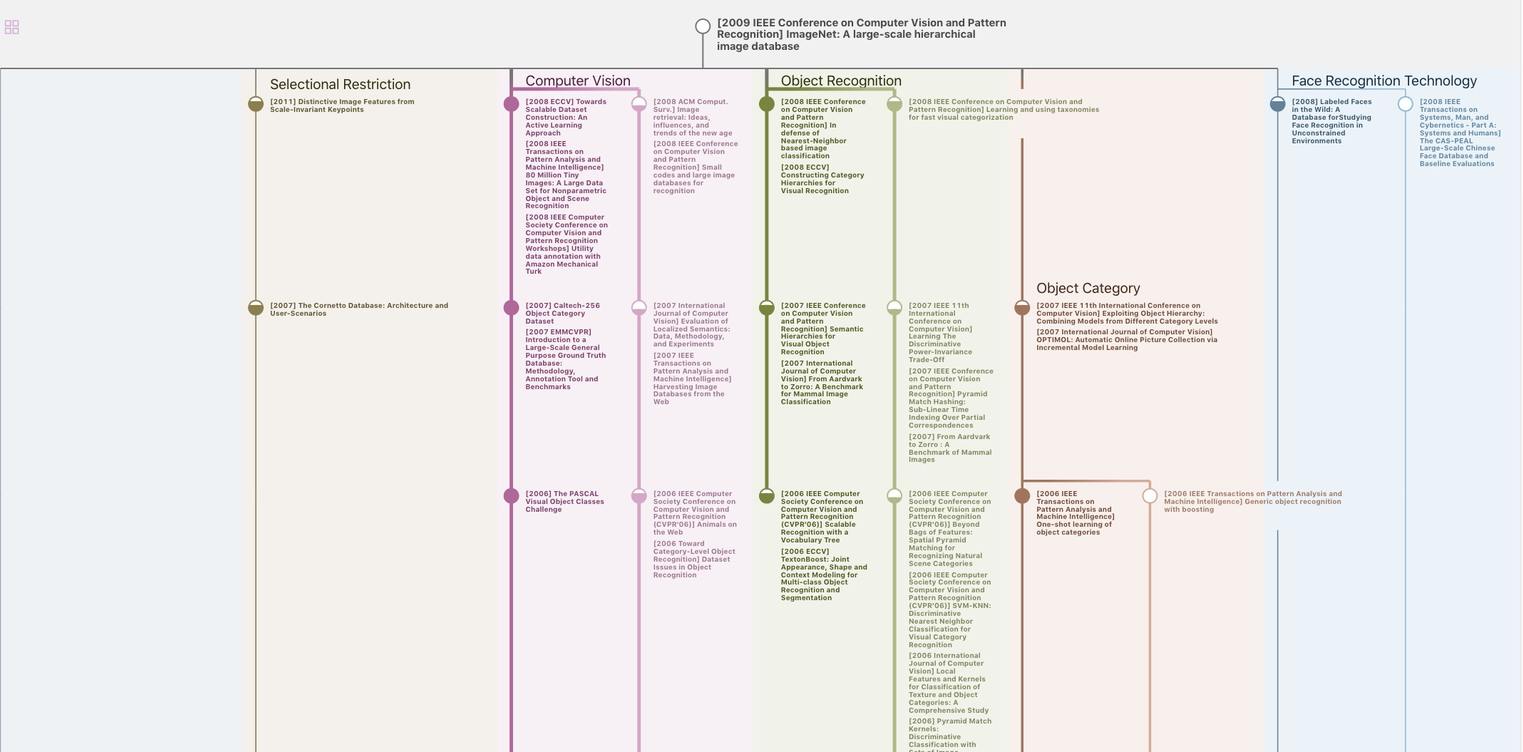
Generate MRT to find the research sequence of this paper
Related Papers
2006
被引用3473 | 浏览
2012
被引用1772 | 浏览
2014
被引用314 | 浏览
2017
被引用359 | 浏览
2018
被引用51 | 浏览
2020
被引用21 | 浏览
2021
被引用2 | 浏览
2022
被引用20 | 浏览
Data Disclaimer
The page data are from open Internet sources, cooperative publishers and automatic analysis results through AI technology. We do not make any commitments and guarantees for the validity, accuracy, correctness, reliability, completeness and timeliness of the page data. If you have any questions, please contact us by email: report@aminer.cn
Chat Paper