A Grading Method of Ginseng (Panax ginseng C. A. Meyer) Appearance Quality Based on an Improved ResNet50 Model
Agronomy(2022)
Abstract
In the academic world, ginseng (Panax ginseng C. A. Meyer) has received much attention as the most representative element of Chinese medicine. To address the lack of traditional algorithms in the identification of ginseng appearance quality and further improve the manual identification on ginseng, we propose a grading method of ginseng appearance quality based on deep learning, taking advantage of the benefits of deep learning in the image identification. Firstly, we substituted LeakyReLU for the conventional activation function ReLU to enhance the predictive power of the model. Secondly, we added an ECA module to the residual block, which allowed attention to be focused on the input object to capture more precise and detailed features. Thirdly, we used the focal loss function to solve the problem of an imbalanced dataset. Then, the self-constructed dataset was processed with data enhancement and divided into four different classes of ginseng. The dataset was trained on a model with transfer learning to finally obtain the best model applicable to the identification of ginseng appearance quality. The experiments showed that, compared with the classical convolutional neural network models VGG16, GoogLeNet, ResNet50 and Densenet121, the proposed model reported the best performance, its accuracy in the test set was as high as 97.39%, and the loss value was 0.035. This method can efficiently classify the appearance quality of ginseng, and has a significant value in the field of ginseng appearance quality identification.
MoreTranslated text
Key words
appearance quality identification of ginseng,deep learning,attention mechanism,activation function,loss function
求助PDF
上传PDF
View via Publisher
AI Read Science
AI Summary
AI Summary is the key point extracted automatically understanding the full text of the paper, including the background, methods, results, conclusions, icons and other key content, so that you can get the outline of the paper at a glance.
Example
Background
Key content
Introduction
Methods
Results
Related work
Fund
Key content
- Pretraining has recently greatly promoted the development of natural language processing (NLP)
- We show that M6 outperforms the baselines in multimodal downstream tasks, and the large M6 with 10 parameters can reach a better performance
- We propose a method called M6 that is able to process information of multiple modalities and perform both single-modal and cross-modal understanding and generation
- The model is scaled to large model with 10 billion parameters with sophisticated deployment, and the 10 -parameter M6-large is the largest pretrained model in Chinese
- Experimental results show that our proposed M6 outperforms the baseline in a number of downstream tasks concerning both single modality and multiple modalities We will continue the pretraining of extremely large models by increasing data to explore the limit of its performance
Upload PDF to Generate Summary
Must-Reading Tree
Example
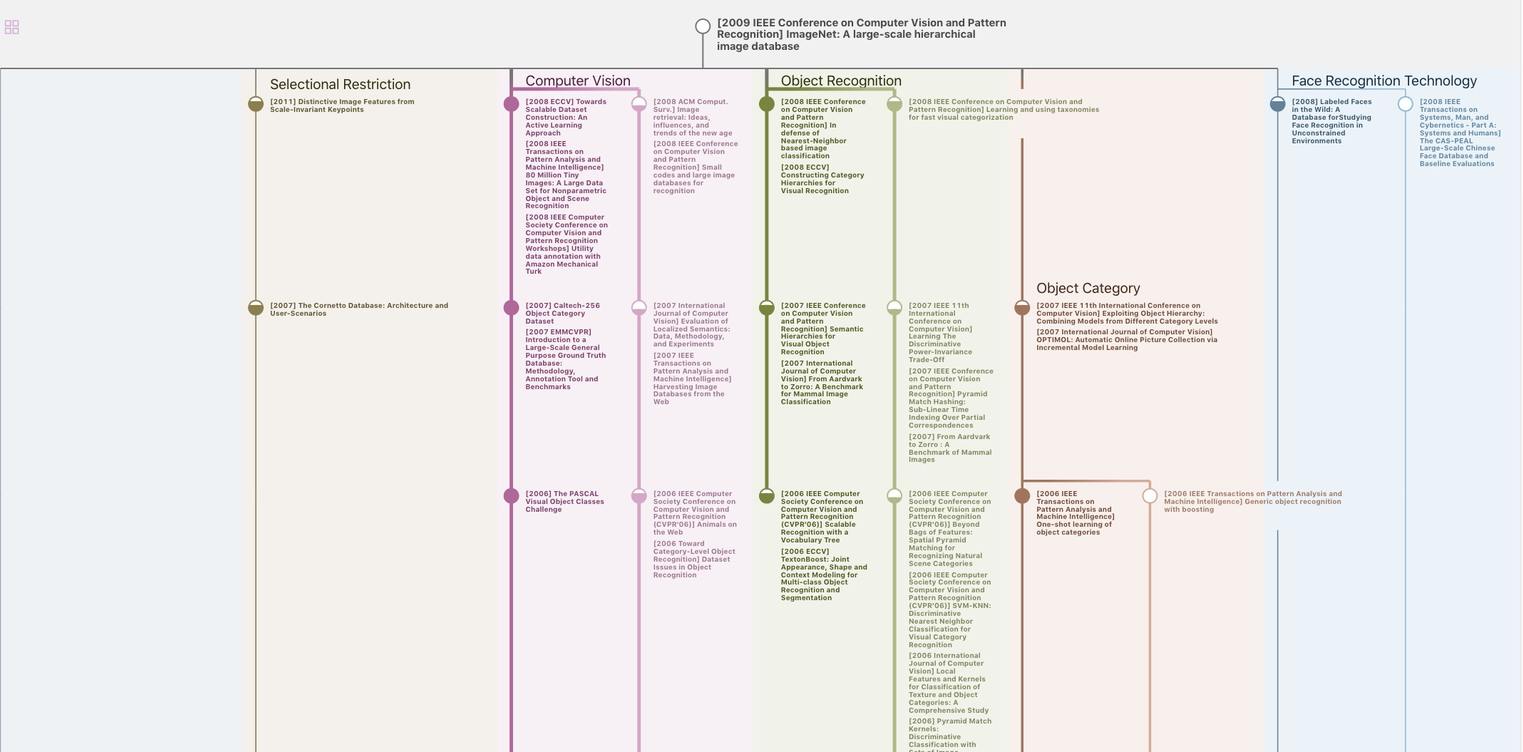
Generate MRT to find the research sequence of this paper
Related Papers
2020
被引用19 | 浏览
Data Disclaimer
The page data are from open Internet sources, cooperative publishers and automatic analysis results through AI technology. We do not make any commitments and guarantees for the validity, accuracy, correctness, reliability, completeness and timeliness of the page data. If you have any questions, please contact us by email: report@aminer.cn
Chat Paper
GPU is busy, summary generation fails
Rerequest